Keys To Success In Six Sigma's 'Measure' Stage
By Steven Zebovitz, R.Ph., Ch.E.
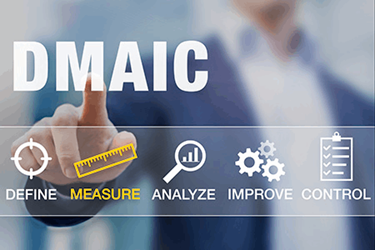
The previous articles in this series provided an introduction to Six Sigma and a discussion of the first stage of the DMAIC process, Define. It would be helpful to review those articles before delving into phase two — Measure.
The Measure phase of a Six Sigma implementation focuses on data collection. Measurements made during this phase must be consistent with the problem definition, collected in a manner consistent with the process map, and inclusive of inputs and outputs. (For more on these topics, see Part 2 of this series.)
Measurements represent both the inputs and outputs of a process or process step. Typical examples of inputs include: raw material characteristics, impurity profiles, temperature, time, revolutions per minute (rpm), dissolved O2, pressure, and power input. Typical examples of outputs are yield, process endpoint readings, and quality release criteria. Where paper batch records are used, measurements consist of hand-documented entries of process operators (including raw material lot numbers). In automated systems, data acquired and logged by SCADA (supervisory control and data acquisition) systems form the data set.
The goal of the Measure phase is generating a “snapshot” of the process at any given time. And, of course, taking measurements across many batches or over a longer period of time constitutes process monitoring. Measurements describe the current process state from which the Six Sigma team must gain a complete understanding. Critical data should follow the process map.
Ideally, data should be
- Unbiased
- Scrupulous in its collection
- Random, except where the data collection plans calls for regular sampling
- Representative
- Reliable and consistent regarding units of measure, time, type, and other defining aspects
- Holistic to the entire process, such as data gained from the suppliers, inputs, process, outputs, and customers (SIPOC) tool
- Accurate
- Precise
The data should be acquired from trained operators, calibrated instrumentation, and well-maintained process and lab equipment.
Measurements are ubiquitous in our industry. Often, there are far more variables documented in our records than are needed to address the project definition. We seek relationships between measurements such that we can equate process inputs to process outputs, known as the X’s and Y’s respectively. In mathematical terms, we are searching for Y = f(X1, X2, X3, …). In the SIPOC format, the goal of data collection is to find the suppliers, inputs, and process data (the X’s) that have the largest effect on the outputs and customers (the Y’s).
Input Variable Types
Data is any variable in the process or process step that can be counted, measured, or otherwise quantified. Numeric data generally falls into the following categories:
- Continuous: Data can take any values within a range, such as 2.33 or 453.6, and are represented by real numbers. For downstream data analyses, continuous data is preferred.
- Discrete: Discrete data is restricted to certain values, such as machine ID numbers, and may fall into the following subsets:
- Count: Examples include acceptable quality limits (AQLs), number of tests, etc. Integers characterize count data.
- Binary: Examples of binary data are on/off, high/low, pass/fail, etc.
- Categorical: These data are characteristics, often descriptive, and consist of non-numeric attributes. They fall into the following categories:
- Nominal attributes: No particular sequence is inferred, and the data is typically non-numeric. Examples include compression department, gender, and account numbers.
- Ordinal attributes: These attributes ascribe an order or ranking to the data, such as poor-medium-good ratings or academic grading (A-F).
Planning for Data Collection
Data collection planning involves the following steps:
- Select the data to collect.
- Decide on operational definitions and stratification factors.
- Determine sample size.
- Identify the source and location of data (including completed batch dossiers and archives if, ideally, the data collection method is understood, recent, establishes a historical pattern, and supplements current data). The process map is the guide for this step.
- Create forms specifying units of measure for data collection (often, batch records require consistent data documentation).
- Decide who collects the data, how its collected, training, and procedures.1
For further description of data collection in a manner that lays the foundation for downstream analysis, see The Lean Six Sigma Pocket Tool Kit.1
Measurement Accuracy
Data is used to make decisions and guide actions, and it will be called upon to analyze defects, variation, process flow, and speed.2 Yet all data has inherent variation. Processes also have variation, so efforts to optimize data accuracy are critical to the success of Six Sigma teams. Calibrations, equipment maintenance programs, periodic equipment performance qualifications, and operator training help ensure measurement accuracy. Also, tracking and trending “as found” calibration data across several calibration cycles can help pinpoint mechanical or electrical issues that require attention.
A key technique for assessing data quality is measurements systems analysis (MSA), such as a gage repeatability and reproducibility (gage R&R) study. A gage R&R is an experiment across multiple variables by multiple operators, and across instruments, sites, etc.3 It is applicable to both continuous and discrete responses, and encompasses random effects, nesting vs. crossed factors, as well as other considerations. The test should be planned and executed prospectively, prior to the process data collection phase.
Note that there are multiple MSAs beyond the gage R&R. These include analyses of bias, stability, discrimination, and kappa (for attribute systems). As part of their data planning and screening activities, the Six Sigma team must consider these studies as well.
Execution of the Measure Phase
The goal of this phase is to ensure that data is collected according to the data collection plan, and that the Six Sigma team has rigorous oversight throughout.
The Six Sigma team should have frequent conversations with operators and other staff members involved. If unexpected or borderline data is obtained (i.e., special cause variation), the team must query operators and other associate members in a priority manner. If no assignable causes for unexpected data are found, then the data is deemed valid and handled according to company policies and good manufacturing practices (GMPs). During downstream data analysis, data must be considered in the context of the process step and product quality.
Completion of the Data Collection Plan
When we arrive at the Analysis step of DMAIC, we will use statistical software packages that analyze data tables consistent with their required formats. Hence, paper records must be transferred into those formats. Data acquired by online systems must be tabulated such that batch-to-batch records are stacked vertically in columns. Remember that GMP decisions will be based on this data. As we preserved the veracity of the data during its collection, we must be equally scrupulous in converting it to data files.
For paper-based systems, data should be converted to data tables and audited by the quality group. This is a cumbersome step, and it is helpful to restrict the conversion to critical data as determined by brainstorming, risk evaluation and mitigation strategies (REMS), etc. If the input X’s that correlate to outputs Y’s are unclear or not known, I recommend that every manual entry be included in the initial data table. If the tables become too unwieldy, then they can be broken into several files, being careful to maintain the data referencing (such as batch numbers).
For SCADA systems, the data must be transferred in a manner that ensures its integrity in a GMP environment and that is consistent with company policies.
Closing Thoughts
Well-planned data collection should proceed almost automatically if the Six Sigma team includes all stakeholders involved in the process. For process data, the input of operators and supervisors closest to the process are critical and often illuminating. For analytical data, chemists and technicians, sample collectors, and sample storage associates must be allowed input.
As I've mentioned in my previous articles, a credentialed and mentored Six Sigma team is vital to the success of this plan. If you haven't already, I urge the readers of these articles to obtain their Black Belts (or Master Black Belts) and gain proper mentorship to help ensure success in process improvement activities.
References:
- George, M.L., et al, The Lean Six Sigma Pocket Tool Kit, The McGraw-Hill Companies, USA (2005), p. 72-74.
- Ibid, p. 8
- Bartell, P., and Hersh, P., Quality and Process Control Methods for Manufacturing and Service Industries, JMP Seminars, SAS, Apr. 2016.
Acknowledgements:
The author thanks his Six Sigma teacher and mentor, Joseph Ficalora (joeficvfr@yahoo.com, 973-727-3788) for his critique of this manuscript. Mr. Ficalora is a Lean Six Sigma Master Black Belt and deployment coach. The author also thanks Joseph Owens (jpomedia1@gmail.com) for his editorial review of this manuscript.
About the Author:
Steven Zebovitz, R.Ph., Ch.E., has three decades of pharmaceutical engineering and manufacturing experience in oral solid dose. Much of his experience is in engineering and manufacturing support, including leadership positions in technology transfer, technical services, process scale-up, validation (PV and CV), and optimization. He led a Six Sigma deployment that yielded 10 Black Belts and one Master Black Belt. His interests include process excellence, lean manufacturing, and championing teams. He may be reached at stevenzebovitz@comcast.net, www.linkedin.com/in/stevenzebovitz/, or 215-704-7629.