AI's Promising Role In Adverse Event Management Of Small Molecule Drugs
By Seema Rajaram, pharmaceutical digital transformation expert
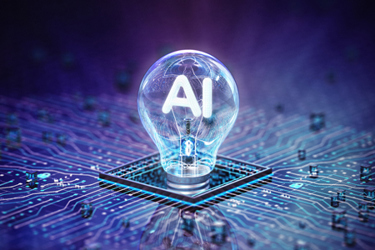
Effective adverse event (AE) reporting is critical in ensuring patient safety and regulatory compliance while manufacturing small molecule drug products. Yet, various limitations impede this process, from reporting deficiencies and data quality issues to fragmented data sources and delayed reporting structures. Compliance complexities, manpower shortages, technical gaps, and data confidentiality concerns further delay reporting, which can lead to potential safety risks and regulatory setbacks. Leveraging AI and data science offers promising solutions, such as automating data gathering, enhancing data quality, and accelerating reporting times. These technologies also can streamline compliance efforts, address confidentiality issues, and improve the robustness of AE reporting systems. By fostering a proactive, technology-driven AE reporting environment, manufacturers can ensure swift, accurate tracking of adverse events — advancing patient safety, meeting compliance standards, and bolstering healthcare quality.
Making AE Reporting More Effective And Efficient
AI and machine learning (ML) can significantly enhance the efficiency and accuracy of AE reporting for small molecule drugs in several ways. Pfizer reported that AI could reduce the time required to process AE reports by up to 70 percent. Another study found that the use of AI-driven chatbots increased patient reporting rates of adverse drug reactions by up to 20 percent.
Say, for instance, that a patient taking Paxlovid experiences an unexpected side effect and uses an AI-powered chatbot to report it. In this situation, the chatbot asks a series of guided questions designed to gather detailed information about the adverse event. The chatbot then analyzes the data in real time, identifying the severity and flagging it for immediate review, if necessary.
The chatbot also advises the patient on managing the symptoms and informs them about the next steps. The collected data is securely transmitted to the pharmacovigilance team and integrated into the broader safety monitoring system. AI-powered chatbots can substantially enhance the efficiency, accuracy, and user experience of adverse event reporting for drugs, ultimately improving patient safety and better pharmacovigilance practices.
Consider the sheer volume of adverse event reports, which can be daunting and create data overload, making it difficult for healthcare professionals and regulatory bodies to process and analyze data promptly and accurately. AI can automate data processing. AI-driven natural language processing can automatically extract pertinent information from large volumes of unstructured data — patient reports, social media posts, and clinical notes. ML algorithms can quickly scan these data sets in search of patterns and anomalies, significantly lightening healthcare professionals’ manual workload.
Adverse event reports on data quality and consistency often come from numerous sources, including healthcare providers, patients, and pharmacovigilance systems. Inconsistencies in the quality and format of these reports can create challenges in integrating and analyzing the data the report yields. AI helps standardize and clean data from numerous sources, such as clinical, safety, and patient databases, putting data into a centralized system in a consistent format that’s easier to integrate and analyze.
ML models can identify and correct data errors or inconsistencies. Data science professionals can do the same, along with spotting missing values. Implementing data standardization protocols helps ensure that data from various sources is consistent and comparable, fostering more accurate analysis.
Chatbots aid accuracy and standardization, guiding users through a uniform reporting process and ensuring that all relevant information is collected consistently. Chatbots also validate data in real time, prompting users to correct any irregularities or incomplete data entries.
Underreporting of adverse events is an ongoing concern in the pharmaceutical industry due to factors such as a lack of understanding of an event’s significance or the sometimes-unwieldy nature of reporting processes. Underreporting, however, can contribute to an inadequate understanding of a drug’s safety profile. AI can enhance reporting mechanisms, with AI-powered chatbots and mobile apps guiding patients and healthcare professionals through the reporting process. Chatbots can proactively contact patients who have been prescribed prescription medicine, reminding them to report any side effects and providing an easy way to do just that. AI systems also can monitor patient health data in real time and prompt users to report unusual symptoms or side effects.
Data science enhances data collection and monitoring, developing predictive models that identify patients or scenarios where underreporting is likely. These models can trigger reminders or alerts to encourage reporting.
Improving Compliance Efforts
Staying in compliance with various regulatory requirements across regions adds a degree of difficulty to AE reporting. Each regulatory body might have its own set of guidelines and timelines for adverse event reporting, making managing reports more complex.
AI automates compliance checks with regulatory algorithms that can be programmed to automatically check adverse event reports for compliance with various regulatory requirements. This ensures timely and accurate submissions. AI also automates the documentation process, generating the documentation needed for regulatory compliance and reducing the administrative burden on staff members.
Data science can automate compliance monitoring, developing rule-based systems to automatically check adverse event reports for compliance with regulatory requirements. Chatbots help ensure regulatory compliance and can be programmed to guarantee that all collected data adheres to regulatory requirements. They also generate and submit the necessary documentation and audit reports to regulatory bodies.
In addition to addressing underreporting and aiding compliance efforts, AI’s capabilities can reduce manual reporting delays. This increases data accuracy for adverse event monitoring by automating data collection and entry, leveraging natural language processing (NLP) for text analysis, performing real-time monitoring and alerts, enhancing data accuracy through consistency checks and error detection, improving data integration, and engaging patients and healthcare providers. The technology also can help streamline adverse event reporting workflows within pharmaceutical enterprises by automating data collection and entry, enhancing data cleaning and preprocessing, improving signal detection and analysis, enabling predictive analytics, automating reporting and compliance, and optimizing resource allocation.
Key Considerations For An Effective Risk Mitigation Plan
Implementing new technologies as part of adverse event reporting processes, however, presents some risks. An effective mitigation plan helps to mitigate these risks and includes components such as:
- Enhanced security measures. Implement robust data encryption, secure authentication, and regular security audits to protect sensitive information.
- Continuous improvement measures. Regularly update and refine chatbot algorithms, using diverse and comprehensive training data to improve accuracy and reduce biases.
- Hybrid approach. Combine AI chatbots with human oversight to handle complex cases and ensure high-quality data collection.
- User education. Educate patients and healthcare providers on the benefits and limitations of AI chatbots to build trust and encourage adoption.
- Inclusive design. Ensure chatbots support a wide range of languages and are accessible to all users, including those with disabilities or limited access to technology.
Ultimately, managing adverse event reports for medications can be complex and challenging. AI and ML can significantly help streamline and improve the process. Additionally, by leveraging AI, pharmaceutical companies can enhance the efficiency, accuracy, and effectiveness of their pharmacovigilance efforts, improving patient safety and drug efficacy.
About The Author:
Seema Rajaram is a global test and validation leader specializing in digital transformation and artificial intelligence within the pharmaceutical industry. With over 18 years of experience in IT, she oversees complex global initiatives, leveraging AI and data science to enhance drug discovery and regulatory compliance processes. Her work focuses on developing and implementing advanced testing frameworks for AI-driven systems, ensuring data integrity, reliability, and operational efficiency. Rajaram also plays a crucial role in risk management and security testing, protecting sensitive patient data and intellectual property in the digital pharmaceutical landscape. Connect with Rajaram on LinkedIn.