Cross-Sector Intelligence: The Prospects For Data Convergence In Biopharma
By Ajay Babu Pazhayattil, Marzena Ingram, and Naheed Sayeed-Desta
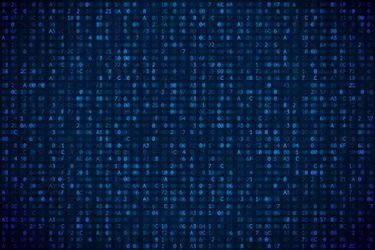
This two-part series of articles looks at how the application of new technologies around data analytics has proven beneficial for the biopharmaceutical industry and regulators alike. The first article explored how technological advancements such as use of real-time data, predictive modeling, and artificial intelligence are gaining regulators’ acceptance and enabling the more efficient development of effective drugs and treatment solutions. This article examines the current environment of collaboration in the industry, along with technologies that will open opportunities to utilize data across life science sectors, including cross sector intelligence (CSI), the convergence of information across multiple sectors, enabling intelligent data-driven decision making.
Era Of Collaboration
Sharing the risks and costs associated with drug discovery while still benefiting from successes is a desirable scenario for the life sciences industry. The current efforts underway to enhance the antibiotic pipeline may point to how the discovery sector should function. According to the Pew road map,1 new antibiotic discoveries have dropped since the 1980s, triggered by diminishing returns on investment in antibiotic research. However, there has been a renewal of interest by drug companies in collaborating with multiple institutions in basic research on antibiotics, with regulators incentivizing the priority discoveries. The new business model includes individual centers of excellence, such as universities for research, pharmaceutical firms for product development, and government agencies for policies, working together to ensure a better success rate in discovery, thereby facilitating accelerated discovery.
Collaboration and sharing of data has become more acceptable to optimize the use of resources and promote better patient outcomes. Numerous biopharma organizations and technology/equipment providers are collaborating with top pharmaceutical university consortiums and research groups, for example, Research Center Pharmaceutical Engineering (RCPE), the Center for Structured Organic Particulate Systems (C-SOPS), and the Novartis-MIT Center For Continuous Manufacturing (CM). These collectives apply machine learning, material information, process information, and scientific literature to develop the best models and tools for the purposes of advancing science. This collaboration enables using cross product/process data effectively to develop the most cost-effective processes, a win-win for industry and patients. Equipment manufacturers have been developing new designs and incorporating new technology for automated monitoring and process adjustment, as this is a necessity for CM and for working with various research groups. Each of the collaborators can focus on their own strengths while delivering the best outcomes. In the CM example, the universities can continue focusing on developing basic CM research, industries will work on scaling the technologies and applying the technology with their individual products, while equipment and technology providers support the user needs.
The trend of such industry and university collaboration is evidenced by a recent announcement by Johnson & Johnson to drive the development of novel solutions that impact healthcare through more than a dozen new collaborations with centers of excellence such as the University of Pennsylvania’s Gene Therapy Program, the Northern California Institute for Research and Education, JLABS, the Koch Institute for Integrative Cancer Research at MIT, Queensland University of Technology, Monash University, and the Taiwan Industrial Technology Research Institute.2 The initiatives are focused on leveraging advances in science and technology, including the use of artificial intelligence, to address areas of high unmet medical need. With such partnerships in place, novel screening and collaboration platforms are necessary. Communication, transfer, and application of data without impact on its integrity is critical for successful outcomes between the industry and institutions, so the need for data integrity, security, and privacy solutions will increase with the growing collaboration in the biopharmaceutical industry.
Enhanced Patient Safety And Compliance
The first drug product with digital ingestion tracking technology is the Abilify MyCite tablet embedded with a sensor that tracks whether the medication was taken.3 The technology is critical for patient adherence since it is indicated for schizophrenia, acute treatment of manic and mixed episodes associated with bipolar disorder, and as an add-on treatment for depression. Patient compliance is very low post placing on a treatment regimen; approximately 50 to 60 percent of patients are likely to skip taking the medications altogether, ultimately resulting in an enormous cost to healthcare.
Estimates show unwarranted deaths of 125,000 patients and a loss of $290 billion annually in the U.S. due to inadequate patient adherence.4 Predictive tools to determine the effects of patient noncompliance for individual drugs can enable tailored interventions. The data aggregation and analysis these tools can provide have generated interest from healthcare insurers and pharmaceutical companies as the information can determine the future product development, product enhancements, and therapy delivery strategies. The analysis and studies have also resulted in development of patient interface monitoring applications, such as the Comprehensive Health Enhancement Support System (CHESS), which provide information, social support, and decision-making assistance to patients dealing with crises.5 The industry also benefits from gaining direct real-world evidence on its products by implementing such tools.
Counterfeiting of drugs has been a major issue affecting patient safety. To protect the supply chain, the Drug Supply Chain Security Act (DSCSA) introduced the serialization or “track and trace” rules. Although it is a tremendous undertaking, multiple parts of the supply chain have been prepared from a technology and data-sharing standpoint to accept the new requirements. The FDA has provided a grace period to implement the associated technologies and processes.6 The solution provides regulatory, logistical, and patient safety benefits, including monitoring of controlled substances, ensuring temperature integrity, provide visibility of supply, preventing delays, and reducing human errors, among others. Predicting drug shortages and ensuring integrity will be possible with the availability of enhanced data on products. The key element is the availability and sharing of data such that the decision making on critical patient aspects can be performed with accuracy. These technologies, the associated data generated, and the interpretations made are improving patient outcomes.
Knowledge Management, Artificial Intelligence, Cross Sector Intelligence
The collection and management of data for critical decision making takes place throughout drug discovery, development, clinical trials, manufacturing, supply and distribution, treatment, usage, and safety monitoring. Accelerating discovery, minimizing bio study impact and cost, as well as optimal process development, are driving needs for the industry since R&D costs are becoming more and more difficult to justify. The industry has therefore started applying data, knowledge management, and data analysis technologies that provide the best outcomes. Multiple platforms and technology solutions are being applied throughout the biopharmaceutical industry. The majority of the solutions use a wealth of source data, including past data and experiences, to make predictions on next steps. More precisely, such solutions focus on making already “intelligent” systems capable of simulating humanlike decision making and execution, enabling those systems to perform functions traditionally achieved by skilled professionals. The system performs tasks normally requiring human intelligence, hence it is known as artificial intelligence (AI).7 The acceptance by the biopharmaceutical industry of operational software and machine learning is increasing, as these can infer the overall operations cost while providing a consistent supply of quality products. The imminent advantage of applying artificial intelligence with increased regulatory acceptance of submission data based on predictive modeling has triggered major biopharmaceutical firms to invest in these kinds of advanced technology applications.
One of the most promising solutions of the 21st century is the implementation of a patient-centric care model allowing use of disparate sources of information to identify treatments that are personalized through AI technology. AI solutions rapidly examine patient files, research, expert opinions, and treatment information to propose treatment methods for clinical application in significantly shorter periods of time. This can be considered disruptive technology, because the current method of diagnosis and treatment recommendation is based solely on physician experience and knowledge, making it subjective and costly.
A study, Comparing Sequencing Assays and Human-Machine Analyses in Actionable Genomics for Glioblastoma,8 approved by multiple institutional boards, provides an insight. The study used IBM’s Watson Genomic Analytics (WGA), a cognitive system developed based on multiple predictive models, to analyze a comprehensive database of multiple sources (integration of multiple data sources), including DrugBank, NCI, COSMIC, ClinVar, 1000 Genomes, and unstructured literature from sources like PubMed. When compared to the efforts of a group of individuals from the bioinformatics, oncology, and various institutions, the WGA system provided superior outcomes from the perspectives of both time and identified root cause. The study revealed that the clinical utility is high, as it identifies drug combinations at a lower cost and in a shorter time. As an AI tool, IBM Watson can supplement clinical decisions, helping to provide effective patient solutions in a timely manner.
Figure 1: Cross-sector intelligence
A key near future aspect is the cross sector utilization of multi-segmented data to make intelligent decisions through use of powerful integrated artificial intelligence tools, such as the one described above. Cognitive software solutions will develop in the biopharmaceutical industry with use of multiple source databases comprising R&D, manufacturing, regulatory, market, and patient data. An example is forecasting future batch sizes of a particular drug product based on information such as patient compliance rate for the drug product, similar category drugs under clinical trials, etc. Algorithms can be developed to predict a physician prescription rate based on the real-world patient adherence data collected. Another example is that of factoring in adverse drug reaction data in developing future product formulation dosage form or delivery system enhancements.
The availability and application of data in a meaningful form for use across sectors can eliminate the costly analyses that biopharmaceutical companies regularly embark upon. This cross sector convergence of intelligence, known as cross sector intelligence (CSI), focuses on creating intelligent decisions based on data sets from multiple sectors. Such intelligence convergence will be a trend for collaborators within the biopharmaceutical industry and beyond. The multitude of knowledge management and artificial intelligence solutions can enable collaboration, particularly enhancing cross institutional and cross company research and advancements. The adoption of artificial intelligence and CSI will open up tremendous potential for opportunities in the biopharmaceutical industry that can provide business success by boosting R&D productivity, improving operational efficiency and predictability, and ultimately ensuring patient safety and drug efficacy.
Note: This article was prepared by the authors in their personal capacity. The opinions expressed are the authors’ own and do not reflect the view of their employer, government, or any agency with which they are affiliated.
References:
- Antibiotic Resistance Project (2016), A Scientific Roadmap for Antibiotic Discovery, PEW
- Meiling, B. (2018), J&J does Alzheimer’s gene therapy deal, along with 14 other new collaborations, Endpoints News https://endpts.com/jj-does-alzheimers-gene-therapy-deal-along-with-14-other-new-collaborations/
- Aungst, T. (2017), What Pharmacists Should Know About the Abilify MyCite Pill, Pharmacy Times http://www.pharmacytimes.com/contributor/timothy-aungst-pharmd/2017/11/what-you-need-to-know-about-the-abilify-mycite-pill
- Hayden , H. B., Granger, B. B., Mendys, P., Brindis, R., Burkholder, R., Czajkowski, S.M., Daniel, J. G., Ekman, I., Ho, M., Johnson, M., Kimmel, S.E, Liu, L.Z., Musaus, J., Shrank, W.H., Buono, E.W., Weiss, K., Granger, C. B (2011), Medication Adherence: A Call for Action, American Heart Journal, Vol 162, Issue 3, Pg. 412-424
- Temesgen, Z, Knappe-Langworthy J. E, St Marie M. M, Smith, B.A, Dierkhising, R.A. (2006) Comprehensive Health Enhancement Support System (CHESS) for people with HIV infection, AIDS and Behavior, Jan;10 (1):35-40.
- Yap, D. (2014), Track-and-trace law: 2015 Deadlines for New Requirements, American Pharmacists Association https://www.pharmacist.com/track-and-trace-law-2015-deadlines-new-requirements
- Marsden, P. (2017), Artificial Intelligence Defined: Useful List of Popular Definitions from Business and Science, Digital Intelligence Today https://digitalintelligencetoday.com/artificial-intelligence-defined-useful-list-of-popular-definitions-from-business-and-science/
- Wrzeszczynski, K. O., Frank, M.O., Koyama, T., Rhrissorrakrai, K., Robine, N., Utro, F., Emde, A., Chen, B., Arora, K., Shah, M., Vacic, V., Norel, R., Bilal, E., Bergmann, E. A., Moore, J. L., Bruce, V., Lassman, A. B., Canoll, P., Grommes, C., Harvey, S., Parida, L., Michelini, V. V., Zody, M. C., Jobanputra, V., Royyuru, A.K., Darnell, R. B. (2017), Comparing sequencing assays and human-machine analyses in actionable genomics for glioblastoma, Neurology Genetics, Aug 01, 2017: 3 (4)
About The Authors:
Ajay Babu Pazhayattil has held key management roles with brand name, generic, and contract manufacturing organizations. He is an industrial pharmacist successful in delivering tangible results across multiple segments of pharmaceutical operations. Ajay has extensive experience in conducting global GMP audits and has been effective in conceiving, implementing, and promoting novel technology innovations based on sound scientific principles. His experience extends through solid-dose, liquids, and small and large volume parenteral dosage forms. Ajay is involved with industry organizations and has published multiple journal articles. Connect with him on LinkedIn.
Marzena Ingram is a pharmaceutical industry professional with extensive quality assurance and technical operations experience; she holds a senior management position. Ingram developed a specialized continued process verification team and spearheaded the implementation of a product life cycle program to meet the global regulatory requirements. She also introduced statistically driven product assessment processes and has been the lead on implementing a comprehensive life cycle management software solution. Ingram also has authored multiple pharmaceutical journal articles. Connect with her on LinkedIn.
Naheed Sayeed-Desta has been responsible for providing strategic directions on life cycle management for over 300 active solid-dose products. Naheed champions delivery of science- and risk-based approaches from traditional to novel manufacturing technologies. She is a proven leader in pharmaceutical manufacturing science and technology. Her expertise in providing pragmatic solutions for manufacturing operations is well recognized. She has been the lead author of journal articles. Naheed is an active contributing member of pharmaceutical industry organizations. Connect with her on LinkedIn.