How AI Tools Will Transform Quality Management In The Life Sciences
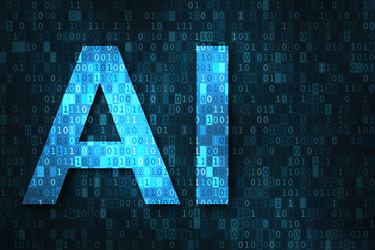
FDA officials and leaders in the pharma and medical device spaces agree artificial intelligence (AI) tools could enable a step change in quality management in those industries. Areas that could be impacted include supply chain management, lot release, manufacturing, compliance operations, clinical trial end points, and drug discovery, among others.
AI has drawn the attention of the pharma industry recently based on impressive successes in other industries, such as machines performing face recognition, driving vehicles, competing at master levels in chess, and composing music. To date, the primary applications of AI in pharma have been in research and development and clinical applications. These include predicting Alzheimer’s disease, diagnosing breast cancer, and precision and predictive medicine applications.
The Xavier Health Artificial Intelligence Initiative brought together key players and experts from industry, academia, and government in August of last year to explore the possibilities and potential roadblocks. At the FDA/Xavier PharmaLink conference in March at Xavier University in Cincinnati, Ohio, two working groups that are part of the initiative gave preliminary readouts of their progress to date. More in-depth summaries will be provided at the Xavier AI Summit in August, along with more detailed discussions of the use of AI in pharma and medical device companies.
The Xavier Health AI Initiative is working to expand on the use of AI across the pharma and device industries. Its task is to identify ways to implement AI across quality operations, regulatory affairs, supply chain operations, and manufacturing operations — augmenting human decisions with AI so decisions are more informed. The vision is to use AI to move the industry from being reactive to proactive, to predictive, and eventually to prescriptive, so actions are right-first-time.
The intent is to increase patient safety by ensuring the consistency of product quality. The initiative aims to promote a move from traditional pharma techniques — such as plant audits and product sampling, which are snapshots in time — to continuous monitoring of huge amounts of GMP and non-GMP data to produce continuous product quality assurance.
What Is AI?
Simply put, AI is shorthand for any task a computer can perform in a way that is equal to or surpasses human capability. It makes use of varied methods such as knowledge bases, expert systems, and machine learning. Using computer algorithms, AI can sift through large amounts of raw data looking for patterns and connections much more efficiently and quickly than a human could.
An AI variant, deep learning, breaks the solution to a complex problem into multiple stages or layers. It examines data sets and discovers the underlying structure, with deeper layers refining the output from the previous ones. A mature system has fully connected layers with both forward and backward comparison abilities.
Another AI subset known as machine learning relies on neural networks — computer systems modeled after the human brain. It involves multilevel probabilistic analysis, allowing computers to simulate and perhaps expand on how the human brain processes information.
Are There Any Red Flags?
As these machines “learn,” the pathways they take to arrive at decisions change, so the original programmers of the algorithms cannot tell how the decisions were arrived at. This creates a “black box” that can be problematic for highly regulated industries such as pharma, where the reasons for decisions and actions need to be documented.
“We are from an industry where we like to validate our processes — it is done once in one way, and we keep doing it that way,” Xavier Health Director Marla Phillips commented at the March conference. “With systems that continuously learn, the algorithm evolves, and it is not the same any more. How do you manage in this new world?”
In addition, since the logic of the decisions is not obvious, decisions the AI machines make might be questioned. The Xavier Health AI Initiative is focused on augmenting human decisions with more robust data and information. The credibility of the data source gives the end user confidence in the outcome.
The process of linking the input to the outcome is referred to as “explainability” and is another work stream Xavier is taking on. Oftentimes, the AI algorithm is considered intellectual property, but through explainability, the end user can know the inputs that led to the outcome. The AI is tested and trained using known inputs and known outcomes first to gain confidence in the algorithm.
This supervised learning is an important first step. Most of the industry is working at this level. However, the next step is unsupervised learning through deep neural networks. Whether supervised or unsupervised, the integrity of the linkage between inputs and the output must be maintained so humans can confidently augment their decisions through AI.
Moving forward, the culture organizations need to have when using AI must be considered. For example, Phillips asked, “What if you get information [from an AI tool] that says, ‘This product is going to fail, do not release it?’ It sure looks the same as is has the past 20 years when we have been releasing it. Are you going to tell your management, ‘Sorry, we have to discard this?’ How do you know this was going to fail? It might be right. We are in a very different decision-making situation and culture.”
A Cautionary Tale
Kumar Madurai, principal consultant and solutions delivery manager at Computer Task Group (CTG) and Xavier AI core team member, provided a cautionary tale regarding trust of the science behind AI-produced decisions, based on an experience with one of his clients.
“One client started off with the idea of having a group dedicated to data analytics. They started in a big-bang way,” he said. “They integrated data from eight different systems. The thinking was that once it was all integrated and linked they could build the queries and the analytics on top of that.
“What happened in that case is they developed some tools. But the subject matter experts [SMEs] who were supposed to take action based on what the tool was telling them did not believe what it was telling them.”
The expertise, training, and culture have to be in place to use AI effectively and confidently. The client decided to start over with simpler tools that had more visualization and capability for more data exploration. It intends to target a system the SMEs will help run, use, and better understand.
Evaluating Continuously Learning Systems
One of the two Xavier AI teams is tasked with exploring how to evaluate a continuously learning system (CLS) — one whose output at different test times may be different as the algorithm evolves. One of the two team leaders for this effort is FDA Center for Devices and Radiological Health (CDRH) Senior Biomedical Research Scientist Berkman Sahiner.
FDA involvement is critical in this effort, as both industry and regulatory agencies need to trust the science behind the AI and evolve their understanding of AI together. In general, industry and regulators have been accustomed to traditional science and validated processes. More information on the team is available here.
The team’s goal is to identify how one can provide a reasonable level of confidence in the performance of a CLS in a way that minimizes risks to product quality and patient safety and maximizes the advantages of AI in advancing patient health. Stated differently, what types of tests or design processes would provide reasonable assurance to a user of a CLS that the output is reliable and useable?
As part of reaching this goal, the team is looking at a series of questions:
- Since a CLS is dynamic, the algorithm changes over time. What are the criteria for updating the algorithm? Is it entirely automated? Or is there human involvement?
- Because the performance changes over time, can we monitor the performance in the field to get a better understanding of where the CLS is going?
- Understanding that users may be affected as the algorithm evolves and provides different responses, what is an effective way to communicate the changes?
- How do we ensure new data that leads to changes in the algorithm is of adequate quality?
The team is also examining the facets of explainability, security, and privacy — exercises important for any new software tool. The CLS team is divided into two sub-teams: one to focus on the pharma aspects, the other on the medical device aspects. The primary deliverable for the CLS team is a white paper covering best practices for a CLS and how they can be adapted into healthcare. It is not intended to be a guidance or standard. The intended audience is medical device software developers and CLS users.
Creating Continuous Product Quality Assurance
A second Xavier AI team is exploring continuous product quality assurance (CPQA) and is also divided into sub-teams looking at the pharma and medical device applications. One of the leaders of this team is Shire Pharmaceuticals Senior VP of Global Quality Technical Operations Charlene Banard, who presented at the March conference at Xavier. More information on the team is available here.
The pharma and medical device industries are familiar with the term “continuous” — for example, continuous process verification (ICH Q8), continued process verification (FDA Guidance on Process Validation), and continuous quality verification (ASTM E2537). However, the CPQA term the team uses is quite different.
The ICH, FDA, and ASTM terms refer to a set of ongoing activities that are documented and transparent. CPQA, on the other hand, will use AI tools including CLSs, which can evolve into a “black box” if the connectivity between the inputs and outputs is not maintained. While learning how to trust those tools is the purview of the CLS team, its work will be critical to ensuring the success of the CPQA effort.
In her presentation, Banard presented the impetus behind her team’s work. “Despite the controls our industry has in place to assure product quality, we still have failures and recalls,” she pointed out. “The assurance of our product quality is limited by the ability of our organization to access, assess, and connect all relevant data, known or unknown, in real time to manage risk, inform decisions, and ultimately enable actions. This team very much believes AI is one of the tools that can change the future.”
Complaints Used As A Model
Noting AI can be “nebulous,” Banard focused the team’s efforts by using a practical model — investigation of a customer complaint related to patient safety. The scope is confirmed safety-related complaints across all types of (bio)pharmaceutical, device, and life sciences products (e.g., drugs, medical devices, combo products).
Complaints were chosen because, “We all have a passion for patient safety and preventing harm. We can have significant impact if we prevent safety-related complaints,” Banard emphasized. In addition, the complaint process is both tangible and familiar to the medical device and pharma industries and can illustrate what data might be valuable to explore using an AI tool to predict future failures.
Complaints are lagging indicators, consisting of an investigation once the problem has already happened. However, Banard said, “If we can look at all the data and systems in a proactive way in the same way we would look at them in a reactive way, we could anticipate and make decisions proactively.”
CPQA Team Deliverables Explained
The CPQA team has three deliverables: process maps, data element models, and definitions of relevant terms (working in concert with the CLS team). Drafts of the CPQA team deliverables are available here.
Initial system maps have been constructed separately for pharma and medical device applications. The maps demonstrate interconnectivity and potential causal relationships between GXP systems, non-GXP systems, and the complaint system. The team recommends the maps include quality system inspection technique (QSIT) and compliance program elements at a minimum and should consider both existing systems and those that do not exist but would be valuable.
Banard provided complaint example system maps (see illustration). Blue boxes represent systems that have data that might be of interest. Grey boxes are data elements that might reside in the two connecting blue boxes.
For example, in the top map, “if you were to do an investigation on a customer complaint, you might take a path to the left, where you are looking in your manufacturing execution system [MES] or your laboratory information management system [LIMS] data. The data elements in between those help structure your data so you can join all the information from those two systems,” she explained. “If you can imagine taking a more linear approach as we can pretty effectively through our fishbone diagrams, you could add to it information gained from an AI tool.”
“If you were able to put an AI tool on top of all of your systems [as shown in the bottom map], the amount of data you could look at for correlations would be quite vast,” Banard pointed out. “That is our objective: to find the correlations that are likely to give us information that will allow us to make predictive or proactive decisions.”
Fishing In The Data Lake
In explaining the team’s second deliverable — data element models — Banard introduced the term “data lake.” A data lake is a combination of all relevant data, including raw data and transformed data, which is used for various tasks including reporting, visualization, analytics, and machine learning. The data lake can include structured data from relational databases; semi-structured data such as CSV files; unstructured data such as emails, documents, and PDFs; and even binary data. Importantly, AI allows the aggregation of information across all systems on an ongoing basis and includes non-GMP systems.
“Envision it as an AI tool being a fisherman, fishing in the data lake,” she said. For example, since “product ID” exists both in the LIMS and enterprise resource planning (ERP) systems, the ability of the AI tool to pull out meaningful data is enhanced.
The data element models deliverable takes the form of a spreadsheet of all in-house systems that contain relevant information for complaint investigations. Also included is data that is not typically in-house, such as geo-political events, patient monitoring, and social media monitoring. A draft of the spreadsheet is available here (under Deliverables). The spreadsheet shows where the same data resides in multiple systems, which enhances the ability to pull out the data in a meaningful way. It is also meant to highlight the concept and value of consistently structured data.
Is Your Company Ready for AI?
In a session on Big Data analytics at the Xavier conference in March, Sight Machine VP of Product and Engineering Ryan Smith looked at how a company can determine if it is ready to successfully bring AI tools in-house. Smith is a member of the Xavier CLS AI team.
Digital readiness, he said, is one thing Sight Machine looks at when examining potential partnerships with customers. It is composed of technical readiness and the state of organizational readiness.
He said organizational readiness is a “far greater predictor of project success than technical aptitude.” On the technical side, there need to be large data sets with “clean data” for AI to be effective. If the data is not clean (i.e., harmonized), the company may spend more time harmonizing the data than using it.
His firm publishes a free-to-use “digital readiness index” (DRI), that “lets you look at your organization or a facility in your organization and assess it on technical readiness — do they have data, what are the systems, etc. — and also organizational readiness — the ability and the culture to change and use some of these tools.”
On the website, companies can find an interactive tool to gauge their readiness for manufacturing digitization and an explanatory DRI white paper. The DRI uses an online questionnaire. Based on the answers to the questionnaire, the DRI uses a weighted scoring system to place organizations into one of five digital readiness zones: connection, visibility, efficiency, advanced analytics, and transformation. For each zone, Sight Machine recommends quick-win projects and areas for investment to develop more advanced capabilities.
As companies move into higher digital readiness zones, they are able to take on projects that can deliver greater impact in operations, quality, and profitability. At lower levels of readiness, project use cases include a global operations view of real-time production across the network and statistical process controls to provide alerts for out-of-control events.
About The Author:
Jerry Chapman is a GMP consultant with nearly 40 years of experience in the pharmaceutical industry. His experience includes numerous positions in development, manufacturing, and quality at the plant, site, and corporate levels. He designed and implemented a comprehensive “GMP Intelligence” process at Eli Lilly and again as a consultant at a top-five animal health firm. Chapman served as senior editor at International Pharmaceutical Quality (IPQ) for six years and now consults on GMP intelligence and quality knowledge management, and does freelance writing. You can contact him via email, visit his website, or connect with him on LinkedIn.