Lost CMC Data In An ALCOA+ World
By Raz Eliav, Beyond CMC
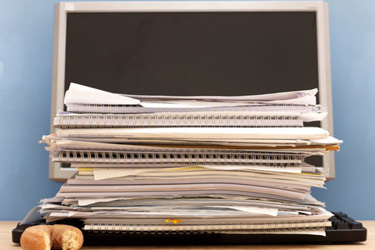
Drug development generates enormous amounts of data. From the biochemistry of a patient's blood in a clinical trial to the sensors of the manufacturing equipment, data is generated continuously and accumulates to petabytes’ worth of gold.
As technologies advance, the products we make are becoming more complex, and so are their data fingerprints. In this article, we will appreciate the vast amount of data that is generated in chemistry, manufacturing, and controls (CMC) development and manufacturing operations of new and existing drugs. We will take a hard look at the way we currently capture and manage this data and see how the document-centric mindset of ALCOA+ is not suitable to support the transition to digital CMC and FAIR data management.
This is the first in a two-part series. In the next article, we will discuss standardization initiatives led by the major regulatory authorities to leverage the current eCTD framework toward a harmonized, HL7-FHIR compliant, digital CMC portfolio.
The Data Elephant
We all know the story of the four blind men describing an elephant. Each one senses a different part and describes what they feel: one describes it as a hard pointy rod, the other as a fluffy rope, and one says it’s a thick, heavy column.
This is our situation when analyzing pharmaceutical products. We are blind in the nanoscale, and each method senses just one aspect of our molecule. The more complex the molecule at hand, the more features that can describe it, thereby creating a complex quality profile.
This is why small molecules can be thoroughly analyzed and described with just a handful of very quick and powerful methods, such as NMR (nuclear magnetic resonance) spectroscopy, infrared, and UV, whereas biologicals comprise much larger entities, with multiple layers of structural features and biological activity. So, instead of describing a few atoms and functional groups, a protein can assume different structural conformations and undergo glycosylation and other post-translational modifications, all with the potential to alter its biological activity and quality profile.
Cell therapies deserve their own paragraph because they increase the complexity by orders of magnitude. As living organisms, they have thousands of discrete structural characteristics (e.g., morphology and surface receptors), as well as metabolic markers, genomes, and protein expression profiles, each of which holds the potential for Big Data analysis.
Feeling dizzy? We are just at the beginning.
The Process Is The Product
Other than the inherent properties of the small molecule or biological product, there lies an additional dimension of variability, especially with biologicals.
There is a saying in biotechnology, “the process is the product” (and vice versa), which implies that the process leaves an inevitable fingerprint on the product. When we manufacture a small molecule, we have a relatively high degree of statistical assurance that under given conditions the resulting product will be as expected. With biological products, any of the abovementioned molecular properties may assume a range of potential outcomes, reacting to slight variations in the manufacturing process. Therefore, with each batch we manufacture, we create a slightly different molecular profile that is reflected (or not) in our batch release data. Throughout development, we make sure that, in spite of the inherent variability, the clinical response remains safe and effective and in fact, we “prove” in development the range of variability within which we consider it clinically insignificant.
Are we missing some connections between the complex array of CMC data and how it translates to the complex array of clinical outcomes? We certainly are. But this is beyond the scope of today’s discussion.
So, our manufacturing history comprises a multi-dimensional array of quality attributes we measure in-process and at release, the actual process parameters and conditions that led to the obtained fingerprint. The more complex the product and/or the process, this array becomes impossible to represent in a simple 2D table that is compatible with 2D papers.
Documents Are For Humans
In a document-centric world, we capture all this vastness in documents. We write protocols and summary reports for any activity (or at least, we should) and we submit documents to the regulatory authorities. Documents are important for human-to-human communication because they give us the context and the narrative we need, alongside the data and its interpretation.
We created an elaborate system of rules so we can trust our documentation practices and ensure data integrity, referred to as ALCOA+. This standard tells us the document and the data inside it are: Attributable, Legible, Contemporaneous, Original, and Accurate, plus Complete, Consistent, Enduring, and Available.
Narratives and documents worked well for us for many years, but with the complexity of today’s pharmaceutical products, data analysis is an integral part of the process, and human-only review is no longer sufficient. Advanced statistical data analysis is oftentimes required to make a review decision, and for that, we must use analysis tools like JMP or Excel, which are oftentimes upstream of the document at hand, with source data not available to the reviewer.
Once the data is presented in a document, retrieving it is painstaking and lengthy, prone to errors and misinterpretation, and, worst of all, oftentimes breaks the ALCOA+ chain.
The Journey Of Data In An ALCOA World
You would imagine that the development team has the easiest access to the raw data, but this is not always the case.
Here is a typical situation in a GxP-regulated ALCOA-minded system: The sponsor (a startup company developing a new drug) is asking their CDMO to send them the product stability data for analysis. The CDMO sends them a scan of a signed paper that was printed with the results, thus complying with ALCOA+.
If the sponsor is lucky, the CDMO will agree to violate their rules and send them the original Excel spreadsheet. They will repeatedly warn the sponsor that this is not QA approved, that they should not be held liable in any form, and that it is “for information only”.
They are right, of course. In a document-centric GxP regulated world, it is of utmost importance to know if the document you are holding is the latest version, if it is correct, etc. So, if they send the Excel sheet, we may very easily lose track of the latest version and the status of the data (is it complete? is it QA verified?). We might accidentally alter the data (no, pharma people don’t always properly lock their Excel sheets), and to make things worse, we might end up (internally) with several versions after running different analyses on each file.
Here is a fictional picture of such a folder:
But we have no other choice. To be able to analyze data we must transfer it back and forth, and documents are simply not a good solution for this. This was the case with paper documents, and it’s still the case with electronic documents.
It is no wonder that we use the term “data mining” to describe the first step we take when we look for answers in our data.
From eCTD To dCTD
The transition from the document-centric principles of ALCOA+ to data management principles such as FAIR is an essential, and significant, paradigm shift the pharma industry must undergo to be on par with almost all other industries.
FAIR stands for Findable, Accessible, Interoperable, and Reusable, meaning that:
- Data must be embedded with its associated metadata to make it possible to Find.
- Data is Accessible through centralized systems and standard communication protocols (think of an iPhone being able to send a text message to Android).
- Data is Interoperable, i.e., it can be universally understood and read by different end users.
- By employing the above three principles, data becomes Reusable, meaning it can be used in different ways for different purposes, and this is exactly what we want our data to be.
Instead of having to look for data according to the document it is written in (i.e., batch record), we will be looking for data in a database. With a structured database and full metadata, finding a specific sensor reading from a specific batch would no longer require digging through hundreds of (hopefully, scanned) pages for a handwritten value. FAIR data can be found very easily by a structured query, and on multiple batches at once. For example: Batch number: All; Process step: 3.2.4; Sensor ID: XYZ; output all readings to a CSV and open in Excel. This could well take weeks in current systems.
In the next part of the series, we will see that the current situation is unacceptable the further you are from the data. Regulators are overworked and underinformed in having to review pharmaceutical data primarily through documents, and they are calling for modernization of the industry. We will discuss initiatives such as the PQ-CMC, which is essentially a first step in “FAIR-ifying” pharmaceutical quality and CMC data and systems like KASA, which will be able to leverage this data and streamline the review process.
These initiatives could well be the emergence of a paradigm shift from having CMC data represented in the electronic CTD to a digital CTD.
About The Author:
Raz Eliav is the founder of Beyond CMC, which is a consultancy that helps startups in drug development leverage the existing knowledge, know-how, and data technologies in reducing development risks, with a focus on the drug quality and manufacturing aspect, known as CMC. Eliav offers strategic consulting in CMC development, operations, and regulatory affairs, and training courses in those realms. He brings over 12 years of experience in all clinical development phases and diverse product modalities, mainly biologicals and advanced therapies.