Model-based Control In Continuous Manufacturing of Biotherapeutics: Technology Enablers
By Anurag S. Rathore, Saxena Nikita, and Garima Thakur
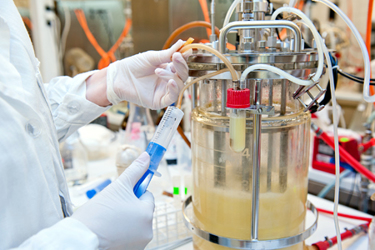
Academic and industrial research groups in the biotherapeutic industry are exhibiting substantial interest in continuous manufacturing.1,2 This has been supported by rapid advancements in technologies that facilitate continuous bioprocessing (Figure 1). One area that continues to be a challenge is that of process control. During continuous processing, automated control techniques coupled with process analytical tools are required to monitor critical quality attributes and implement real-time control decisions to handle deviations.3-5 In this two-part article series, we elucidate how model-based control can be effectively implemented in the various unit operations to offer integrated continuous control.
Figure 1: Various enabling technologies for different unit operations. Click on figure to enlarge.
Figure 2: Overview of model development
Prerequisite For Model-based Control
In any process life cycle, knowledge generation, monitoring, and control are critical for handling uncertainties and feed variability during processing. Model-based control can be quite effective in managing these challenges.7 A process model is basically a correlation between the critical quality attributes (CQAs) and critical process parameters (CPPs) and raw material attributes (Figure 2). Modelling of biotech unit operations is, however, challenging due to the complexity of the underlying physicochemical processes. As the models follow different paths dynamically, either oversimplified models (due to the multiple assumptions) or highly complex models are often developed. It is necessary to take into account the effects of process non-linearity, uncertainty, delays, interactions, unpredictability, and non-reliable intra/intercellular variable measurements. In combination with real-time measurements, model-based methods can be used as soft sensors and act as effective process monitoring tools. Here, proper application of inline sensors and process analytical tools and communication between different unit operations and software units are crucial.
In addition, process models can be used to determine the optimal operating conditions of the process. However, multi-objective optimization can be computationally extensive, and the success depends on the quality of the model. Also, application of model-based methods as data mining tools requires large data repositories and communication over a wide range to access the data from anywhere.
Model-based Methods For Different Unit Operations
Upstream bioreactor: The bioreactor, where the product of interest is produced, is the foremost operation of bioprocessing. Starting by understanding the rate of cell growth to rate of cell death and cell lysis is critical. Over the past several decades, researchers have proposed a variety of process models for this unit operation, ranging from mechanistic models based on mass balance7,8 to statistical models based on neural networks.9,10 However, it is usually an arduous task to develop an elaborate bioreactor model with high prediction efficiency and the capability to fully explain the process phenomenon. Model-based controllers have been demonstrated for achieving high product concentration11 and for controlling dissolved oxygen.12,13 Another challenge is the high computational time required to run the model repeatedly. However, with innovations in spectroscopy (mass/dielectric/Raman/near infrared/Fourier transform infrared), dye-based methods, fluorescence-activated cell sorting, and filtration biosensors, understanding cell function has become a little more accessible.14,15 As continuous processing gets implemented, model-based control of bioreactors will become commonplace.
Continuous capture chromatography: Process chromatography is a key downstream unit operation for capturing target protein and removing aggregates with closely related variants. Performing this operation in continuous mode can be challenging owing to the complex integrated setup of multiple columns and managing titer variations and quality attributes without being a bottleneck for downstream processes.16,17 One of the potential solutions for control is to schedule chromatography steps by integrating multiple columns, inlets, outlets, and valves concurrently. This involves using periodic multicolumn switching methods to enable chromatography steps with continuous switching between columns. Several commercial continuous chromatography systems, such as Cadence BioSMB (Pall Life Sciences, USA), Akta PCC 75 (GE Life Sciences, Sweden), BioSC Lab (Novasep Inc., France), and Contichrom’s (ChromaCon AG, Switzerland) multicolumn countercurrent solvent gradient purification (MCSGP)system, are based on this approach. A unique challenge of this unit operation comes from the fact that Protein A resin, the de facto choice for this step, happens to be the most expensive resin in the entire process and the biggest contributor to the downstream processing cost.18,19 Therefore, a need to optimize resin utilization for maximum usage by loading up to dynamic binding capacity (DBC) arises.20 As a way out, to predict DBC and breakthrough curves, mechanistic models based on general rate models (GRMs) have been proposed for designing and optimizing multicolumn chromatography operation. Multivariate approaches are also being implemented for monitoring column health and fouling.16,21 The dependency of DBC on feed titer creates additional complexity, as feed titer varies significantly during the perfusion process as well as with column residence time and flow rate.16,22
Continuous polishing chromatography: Contrary to capture chromatography where the entire elution peak is collected during the process, polishing chromatography requires precise elution collection points, as this column is the primary step for managing both size-based heterogeneity (aggregates) and charge-based heterogeneity (acidic and basic variants). Since there will be variability in feed composition during processing, real-time control of elution pooling is a major challenge in continuous processing. It requires sensitive decision-making skills to match CQA profiles of each elution pool to the specified benchmark.1,5,23,24 Implementing real-time pooling decisions is difficult due to phenomena like degradation in column performance with use, variation in titer as well as feed quality, or equipment error over long time intervals.25,26 Mechanistic models based on GRM have been used for real-time model-based control of pooling.27,28 In some scenarios, integrated multiple polishing chromatography steps have been proposed. For example, anion exchange followed by cation exchange or cation exchange followed by hydrophobic interaction chromatography have been demonstrated to be active.29
Continuous viral inactivation: Viral inactivation involves virus denaturing that can contaminate the product.30 The most common method employed for viral inactivation is low pH hold performed after Protein A chromatography.31,32 The process is challenging because the nature of the virus might vary from application to application and the same residence time may not hold true for all. If pH is not adjusted correctly, CQAs can be adversely affected.30 Thus, it is critical to maintain proper hold time as well as pH for each cycle. Various enabling technologies for continuous operation involve using a tubular/coiled flow reactor, 32-35 an automated multivessel system,36 or an additional chromatography column.37,38 Model-based control of viral inactivation during continuous process is yet to be demonstrated.
Continuous precipitation: Precipitation, an alternative to chromatography, is a simple and cost-effective method for separation in biotherapeutics manufacturing.39,40 The operation is conducted in batch mode either by precipitating impurity41 or by precipitating product.42,43 Both approaches have their own merits and demerits. In batch mode, the material is placed in the hold tank, where the reactants are added for precipitation and solids are removed.44 However, in continuous mode, consistent monitoring of the addition of reactant and progress of the reaction is required.45 Recently published reports on real-time control of processes involving mixing and reaction could elucidate the approach that one can take for controlling continuous precipitation.46,47
Continuous filtration: Regulatory agencies across the globe are demanding that biotherapeutic products meet stringent formulation requirements. As part of the quality target product profile, careful monitoring of CQAs such as protein and excipient concentrations is required.48,49 Tangential flow membrane ultrafiltration is often used for this purpose; it consists of multiple ultrafiltration (UF) steps to concentrate product to the specified final concentration and a diafiltration (DF) step to exchange the process buffers with the final formulation buffer to impart stability and osmotic balance to the product.50-52
During the operation, excipient concentration drift creates complexity in its control. It is often caused during high concentration ultrafiltration due to electrostatic interactions. With increasing popularity of high concentration subcutaneous drug formulations, overcoming this challenge is of extreme importance. An effective model-based control that has been suggested is to manipulate the composition of the DF buffer.46 Multiple mechanistic models based on Poisson-Boltzmann or Nernst-Planck equations for mass transport of charged particles across a selectively permeable membrane have been proposed for simulating excipient drift in high concentration UF. 46,53-56 However, extensive data and expertise are required to calibrate the model parameters. The process model is highly specific, as changing membrane type, module shape/size, flow rate, pressure, and nature of the feed necessitates recalibration of model parameters.57-60 In addition, process uncertainty and deviations are not accounted for by the model. Therefore, process analytical tools combined with mechanistic models are a preferrable solution.
As an alternative to traditional batch mode tangential flow filtration (TFF), single pass TFF (SPTFF) is gaining popularity. SPTFF, a combination of multiple TFFs in series, is a continuous mode of operation consisting of a long flow path with single pass. It enables low shear stress, eliminates in-process recirculation tanks, produces shorter process times, and provides the ability to integrate with continuous train and high volumetric concentration factors. For a single membrane of a SPTFF module, the rate of change of permeate flux over time is modelled to optimize the process.61 Along with the ample benefits the equipment offers, control and monitoring is onerous. Recent studies suggest using an approach for output concentration control of continuous ultrafiltration in case of perturbations in feed volume or concentration.61 The approach also takes into consideration the different cycle times of different unit operations.
Conclusion
Modelling and expert system application are crucial to facilitate the shift from batch to continuous bioprocessing. Novel model-based control strategies assist stable process operation and improve efficiency. Additionally, they support process integration, control strategy development, and regulatory requirements, thereby facilitating achieving consistency in product quality. However, owing to the complexity of the bioprocesses, it is often challenging to integrate different models for monitoring and control applications. This article discusses the recent technologies developed to run the bioprocess unit operation in a continuous manner. In the next part of the series, we will discuss process integration and control in the context of continuous processing.
References
- Konstantinov, Konstantin B, and Charles L Cooney. “White paper on continuous bioprocessing. May 20-21, 2014 Continuous Manufacturing Symposium.” Journal of pharmaceutical sciences vol. 104,3 (2015): 813-20. doi:10.1002/jps.24268
- Rathore, Anurag S., Continuous processing for production of biopharmaceuticals. Preparative Biochemistry and Biotechnology, 45, 8 (2015), 836-849. doi:10.1080/10826068.2014.985834.
- Rathore, Anurag S, and Winkle H, “Quality by design for biopharmaceuticals,” Nat Biotechnol, vol. 27, 1 (2009), pp. 26–34
- Rathore, Anurag S., et al. “Process analytical technology (PAT) for biopharmaceutical products” Analytical and Bioanalytical Chemistry, 398, 1 (2010), 137–154.
- Glassey, J., et al. “Process analytical technology (PAT) for biopharmaceuticals” Biotechnology Journal, 6, 4(2011), 369.
- Kroll, P., et al. “Model-Based Methods in the Biopharmaceutical Process Lifecycle” Pharm Res 34, (2017) 2596–2613. https://doi.org/10.1007/s11095-017-2308-y
- Kumar, S V Sunil et al. “Nonlinear control of bioreactors with input multiplicities--an experimental work.” Bioprocess and biosystems engineering vol. 28,1 (2005): 45-53. doi:10.1007/s00449-005-0014-3
- Junker, B H, and H Y Wang. “Bioprocess monitoring and computer control: key roots of the current PAT initiative.” Biotechnology and bioengineering vol. 95,2 (2006): 226-261. doi:10.1002/bit.21087
- Sivakumaran, N, et al. “Identification and control of bioreactor using recurrent networks,” Instrumentation Science and Technology, vol. 34, 6 (2006), pp. 635–651, 2006.
- Nagy, Z K, “Model based control of a yeast fermentation bioreactor using optimally designed artificial neural networks,” Chemical Engineering Journal, vol. 127, 1–3 (2007), pp. 95–109
- Ashoori, A, et al. “Optimal control of a nonlinear fed-batch fermentation process using model predictive approach” J. Process Control 19, 7 (2007) 1162–1173.
- Gomes, J. and Menawat, A. S. “Precise control of dissolved oxygen in bioreactors – a model-based geometric algorithm”. Chem. Eng. Sci. 55, 1 (2000), 67–78.
- Nayak, R. and Gomes, J. (2009). Sequential adaptive networks: an ensemble of neural networks for feed forward control of L-methionine production. Chem. Eng. Sci. 64 (10): 2401–2412.
- High-throughput next-generation sequencing technologies foster new cutting-edge computing techniques in bioinformatics
- Zhang, Zhaorui et al. “High-throughput proteomics.” Annual review of analytical chemistry (Palo Alto, Calif.) vol. 7 (2014): 427-54. doi:10.1146/annurev-anchem-071213-020216
- Bangtsson, P., et al. “Method in a chromatography system”. US Patent No. US20120091063A1, GE Healthcare Bio-Sciences AB. (2012)
- CMC Biotech Working Group. “A-Mab: a case study in bioprocess development”. In CASSS, Emeryville (https://casss.org/associations/9165/files/A-Mab_Case_Study_Version_2-1.pdf. (2009).
- Pathak, M., & Rathore, Anurag S. “Implementation of a fluorescence based PAT control for fouling of protein A chromatography resin” Journal of Chemical Technology & Biotechnology, 92, 11 (2017), 2799-2807.
- Pathak, M., et al. “Re-use of Protein A Resin: Fouling and Economics” BioPharm International, 28, 3 (2015).
- Hober, S., et al. “Protein A chromatography for antibody purification”. Journal of Chromatography B, 848, 1 (2007), 40-47.
- Chmielowski, R. A, et al. “Definition and dynamic control of a continuous chromatography process independent of cell culture titer and impurities” Journal of Chromatography A, 1526, (2017), 58-69.
- GE Healthcare. (2005). MabSelect SuRe—studies on ligand toxicity, leakage, removal of leached ligand, and sanitization.
- Flatman, S., et al. “Process analytics for purification of monoclonal antibodies”. Journal of Chromatography B, 848, 1 (2007), 79-87.
- Schiestl, M., et al. “Acceptable changes in quality attributes of glycosylated biopharmaceuticals” Nature biotechnology, 29, 4(2011), 310-312.
- Rathore, Anurag S., et al. “Case study and application of process analytical technology (PAT) towards bioprocessing: Use of on‐line high‐performance liquid chromatography (HPLC) for making real‐time pooling decisions for process chromatography” Biotechnology and bioengineering, 100, 2(2011), 306-316.
- Tiwari, Anamika “Use of HPLC as an Enabler of Process Analytical Technology in Process Chromatography”, Analytical Chemistry 90, 13(2018), 7824-7829, DOI: 10.1021/acs.analchem.8b00897
- Kumar, V, & Rathore, Anurag. S, “Mechanistic Modeling Based PAT Implementation for Ion-Exchange Process Chromatography of Charge Variants of Monoclonal Antibody Products” Biotechnol J., 12, 9(2017)
- Shekhawat, Lalitha. K, et al. “Application of CFD in Bioprocessing: Separation of mammalian cells using disc stack centrifuge during production of biotherapeutics”. J. Biotechnol. 267 (2018), 1–11.
- Carta, G., & Jungbauer, A. Protein chromatography: process development and scale-up. John Wiley & Sons. 2020
- Shukla, A. A., & Aranha, H. (2015). Viral clearance for biopharmaceutical downstream processes. Pharmaceutical Bioprocessing, 3(2), 127–138. https://doi.org/10.4155/pbp.14.62
- Shukla, A. A. et al. Downstream processing of monoclonal antibodies—Application of platform approaches. Journal of Chromatography B, 848, 1(2007), 28–39. https://doi.org/10.1016/j.jchromb.2006.09.026
- Klutz, S., et al. “Continuous viral inactivation at low pH value in antibody manufacturing”. Chemical Engineering and Processing: Process Intensification, 102 (2016), 88-101. doi:10.1016/j.cep.2016.01.002.
- Gillespie, C., et al. “Continuous In‐Line Virus Inactivation for Next Generation Bioprocessing” Biotechnology Journal, 14, 2 (2018), 1700718. https://doi.org/10.1002/biot.201700718
- Kateja, Nikhil, et al. “A novel reactor configuration for continuous virus inactivation” Biochemical Engineering Journal, 167 (2021), 107885. https://doi.org/10.1016/j.bej.2020.107885
- Parker, S. A., et al. “Design of a novel continuous flow reactor for low pH viral inactivation”. Biotechnology and Bioengineering, 115, 3(2017), 606–616. https://doi.org/10.1002/bit.26497
- Schofield, M., & Johnson, D, “Continuous Low-pH Virus Inactivation: Challenges and Practical Solutions” Genetic Engineering & Biotechnology News, 38, 15(2018), S13–S15. https://doi.org/10.1089/gen.38.15.12
- Senčar, J., et al. “A narrow residence time incubation reactor for continuous virus inactivation based on packed beds” New Biotechnology, 55 (2020), 98–107. https://doi.org/10.1016/j.nbt.2019.10.006
- Bolton, G. R., et al. “Inactivation of viruses using novel protein A wash buffers” Biotechnology Progress, 31, 2(2014), 406–413. https://doi.org/10.1002/btpr.2024
- Stavrinides, S., et al. “Effects of engineering parameters on the precipitation, recovery and purification of proteins, in: Ayazi Shamlou, P. (Ed.). Processing of Solid‐Liquid Suspensions, Butterworth‐Heinemann (1993), pp. 118–158.
- Capito, F., et al., Feasibility study of semi‐selective protein precipitation with salt‐tolerant copolymers for industrial purification of therapeutic antibodies. Biotechnol. Bioeng. (2013), 110, 2915–2927.
- Kumar, A., et al. Precipitation of proteins: nonspecific and specific, in: Kaul, R. H., Mattiasson, B. (Eds.). Isolation and Purification of Proteins, Marcel Dekker 2003, pp. 225–276.
- Wang, J. M., et al. Precipitation of process‐derived impurities in non‐Protein A purification schemes for antibodies. Biopharm Int. 2009, Suppl., 1–6.
- Kuczewski M, et al. “A single‐use purification process for the production of a monoclonal antibody produced in a PER. C6 human cell line” Biotechnol. J. 6 (2011), 56–65.
- Hammerschmidt, N., et al. “Continuous precipitation of IgG from CHO cell culture supernatant in a tubular reactor”. Biotechnol. J., 10 (2015), 1196–1205.
- Kateja, Nikhil, et al. “Continuous precipitation of process related impurities from clarified cell culture supernatant using a novel coiled flow inversion reactor (CFIR)” Biotechnology Journal, 11, 10 (2016), 1320–1331. https://doi.org/10.1002/biot.201600271
- Hebbi, Vishwanath et al. “Process Analytical Technology implementation for peptide manufacturing: Cleavage reaction of recombinant Lethal Toxin Neutralizing Factor (rLTNF) concatemer as a case study” Analytical Chemistry. 92, 8(2020), 5676–5681
- Hebbi, V., et al. “Process analytical technology application for protein PEGylation using near infrared spectroscopy: G-CSF as a case study. Journal of biotechnology”, 325 (2021), 303–311. https://doi.org/10.1016/j.jbiotec.2020.10.006
- Le Basle, Y, et al. “Physicochemical Stability of Monoclonal Antibodies: A Review”. Journal of Pharmaceutical Sciences 109 (2020), 169-190
- Shire, S.J., “Formulation and manufacturability of biologics”. Current opinion in biotechnology 20(2009), 708-714
- Ahrer, K., et al. “Effects of ultra-/diafiltration conditions on present aggregates in human immunoglobulin G preparations”. Journal of membrane science 274 (2006), 108-115.
- Shultz, J.E., et al. “Quality by Design as Applied to Drug Substance Formulation Using Ultrafiltration and Diafiltration”, Quality by Design for Biopharmaceutical Drug Product Development. Springer, (2015) pp. 191-210
- Van Reis, R. and Zydney, A., “Bioprocess membrane technology”. Journal of Membrane Science 297, (2007), 16-50.
- Miao, F. et al. “Theoretical analysis of excipient concentrations during the final ultrafiltration/diafiltration step of therapeutic antibody”, Biotechnology progress, 25 (2009) 964-972
- Steele, A. and Arias, J. “Accounting for the Donnan effect in diafiltration optimization for high-concentration UFDF applications”, BioProcess Int, 12 (2014) 50-54.
- Stoner, M.R, et al. “Protein− solute interactions affect the outcome of ultrafiltration/diafiltration operations”. Journal of pharmaceutical sciences 93 (2004), 2332-2342
- Teeters, M., et al. “Predicting diafiltration solution compositions for final ultrafiltration/diafiltration steps of monoclonal antibodies”. Biotechnology and bioengineering 108 (2011), 1338-1346
- Binabaji, E., et al. “Theoretical analysis of the ultrafiltration behavior of highly concentrated protein solutions”, Journal of membrane science, 494 (2015), 216-223.
- Hepbildikler, S., et al. Variable tangential flow filtration. Google Patents (2014)
- Hung, J.J., et al. “High concentration tangential flow ultrafiltration of stable monoclonal antibody solutions with low viscosities”, Journal of Membrane Science, 508 (2016) 113-126.
- Shire, S., Monoclonal antibodies: meeting the challenges in manufacturing, formulation, delivery and stability of final drug product. Woodhead Publishing. 2015
- Thakur Garima and Rathore Anurag S. “Modelling and optimization of single-pass tangential flow ultrafiltration for continuous manufacturing of monoclonal antibodies”, Separation and Purification Technology, Volume 276 (2021), 119341, https://doi.org/10.1016/j.seppur.2021.119341.
About The Authors:
Anurag S. Rathore, Ph.D., is an Institute Chair Professor at the Department of Chemical Engineering, Indian Institute of Technology, Delhi, India. He is also the coordinator for the Centre of Excellence for Biopharmaceutical Technology and is presently serving as IIT Delhi’s Dean of corporate relations. His previous roles included management positions at Amgen Inc. and Pharmacia Corp. His areas of interest include process development, scale-up, technology transfer, process validation, biosimilars, continuous processing, process analytical technology, and quality by design. He has authored more than 500 publications and presentations in these areas. He is presently serving as the Editor-in-Chief of Preparative Biochemistry and Biotechnology and Associate Editor for Journal of Chemical Technology and Biotechnology. He has a Ph.D. in chemical engineering from Yale University.
Saxena Nikita is research associate working under Prof. Anurag Rathore at Indian Institute of Technology, Delhi. She is an expert in process control and is presently working on automation and digitization of biopharma processes for continuous production of biopharmaceuticals. Her areas of interest include process integration, model-based control, statistical process control, and implementation of multivariate data analytical techniques for process monitoring and control. She has published more than 20 articles and a book related to control of various processes, and has filed two patents in the biopharmaceutical field.
Garima Thakur is a researcher working under Prof. Anurag Rathore in the Bioprocessing Lab at the Indian Institute of Technology, Delhi. She works in the area of PAT applications for continuous downstream manufacturing of biopharmaceuticals and has authored more than 10 publications and four patent filings in the field. Her work is focused on overcoming the unique challenges associated with continuous operation in the biopharma industry, particularly handling process deviations with real-time control tools. She has worked on developing novel PAT tools, including data-driven AI/ML solutions and novel spectroscopic tools for improved control of unit operations in the continuous downstream manufacturing train.