Next-Gen Bioinformatics: The Expanding Role Of Deep-Learning Algorithms
By Jen Brown, Thermo Fisher Scientific
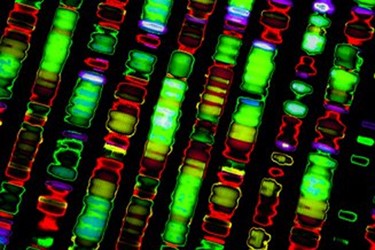
Deep-learning algorithms have shown significant promise as applications in natural language understanding, decision making, and speech and image recognition. These algorithms are now being applied in bioinformatics applications within the biopharma industry to manage the increasing amounts of data from high-throughput techniques. As a bioinformaticist, I am particularly fascinated with recent applications of these algorithms to predict a variety of biological processes and interactions, particularly with respect to proteins.
Many methods exist to identify novel protein-protein interactions (PPIs), but they only contribute to a small percentage of the whole PPI database due to low efficacy. Researchers at the Center for Quantitative Biology in Beijing have now applied a deep-learning algorithm to sequence-based prediction of human PPIs, the best model of which had an average training accuracy of 97.19%. Overall, the predictive accuracies for diverse external datasets ranged from 87.99% to 99.21% and showed promise in other species.
PPIs and the Need for High-throughput Computational Methods
Most proteins interact with other proteins in order to function properly, and thus should be studied in the context of those interactions to fully understand their function. PPIs are known to play a critical role in many biological processes including signal transduction, protein folding, cellular organization, and immune response. Transient PPIs are expected to control the majority of cellular processes and are expected to be involved in the entire range of cellular processes.
As a result, the analysis of PPIs may shed light on drug target detection and aid in therapy design. There are many methods that are commonly used to analyze PPIs ranging from co-immunoprecipitation (co-IP) for stable or strong PPIs to crosslinking protein interaction analysis for transient or weak PPIs. Advances in high-throughput technology such as mass spectrometric protein complex identification (MS-PCI) and yeast two-hybrid screens are capable of generating copious amounts of data but tend to be expensive, time consuming, and may not be applicable to proteins from all organisms. This has led to the need in the industry for high-throughput computational methods to identify PPIs with high quality and accuracy.
Get unlimited access to:
Enter your credentials below to log in. Not yet a member of Pharmaceutical Online? Subscribe today.