Optimizing Digitalization To Accelerate Your R&D
By George Marinos and Mark Humphries, PA Consulting
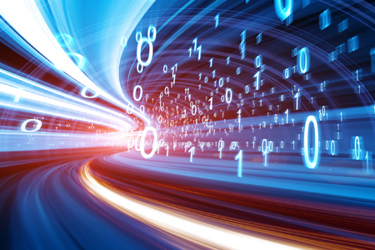
The size of the drug discovery market is expected to grow by over 9% CAGR from 2023 to 2030, reaching $120 billion by 2030. This growth is being driven by a number of factors, including the rising cost of treating an aging population that lives longer, the opportunities enabled by new advanced personalized therapies, the need to mitigate patent cliffs, and the drive to meet unmet clinical needs.
This has heightened demand across pharma and biotech organizations to innovate and launch more new products that meet an increasingly complex and segmented patient market faster and with a greater success rate. It is now common to see R&D pipelines chasing the next blockbuster therapy and tying up significant R&D investment on fewer, larger medicines. There is also a growing need to increase the efficiency of taking drugs to market so a larger portfolio of drugs with lower annual volumes can be delivered cost effectively. A key driver is to transition products from R&D into commercial manufacturing operations more smoothly and achieve optimum manufacturing output to meet patient demand and commercial success.
Drug development has made significant leaps in innovation in recent years with the introduction of personalized therapies and new treatments for previously incurable or complex diseases. The use of robotics and automation in labs has improved the quality and operational efficiency of the drug discovery and development processes. Furthermore, pharma companies have been scaling their data analytics capabilities and applying artificial intelligence (AI) and machine learning (ML) through manufacturing and operations for several years. The success of data analytics in manufacturing coupled with significant developments in data management has led pharma companies to recognize the value in leveraging this capability and applying it more extensively in R&D to create a seamless transition between R&D and manufacturing. The potential value is significant, especially if data analytics can be harnessed to improve the success rate of the drug development and improve speed and predictably of scale-up and release. The benefits of data analytics have also been recognized by regulatory bodies such as the FDA, which, with passage of the FDA Modernization Act 2.0, now allows more extensive use of computer models.
Yet, a number of common issues exist across the drug development process that hinder progress. Systems, processes, and ways of working are not connected and result in labor-intensive iterative tasks that can cause costly delays throughout all phases of drug development and transition into manufacturing. R&D and chemistry, manufacturing, and controls (CMC) priorities and processes are often focused on delivering specific discrete outcomes rather than being aligned to optimize the end-to-end goal; particularly useful data that could support downstream stage gates is not always captured by upstream processes. The use of data follows similar patterns; complex legacy architectures are not integrated across multiple software platforms, resulting in data being held across different systems. In addition, physical pilot platforms used during R&D differ from existing manufacturing assets, which impacts the transition to manufacturing and creates issues when scaling up.
The question is, can a shift toward data-led drug development enable pharma companies to achieve the required leap in productivity? And what do pharma companies need to do to achieve it?
To realize this opportunity, pharma companies must start by considering what data is required, how to capture and store it, what translation is needed, and how to embed this across the chemistry and manufacturing processes. The typical outcomes pharma companies would strive for are greater accuracy of molecular and process simulations, increased process automation, improved process control, and measurement across the value chain. Data-led drug development will, in turn, increase collaboration across critical functions and enable engineers, scientists, quality, and manufacturing experts to develop transformative use cases that aim to reduce lead times, reduce overall development cost, and improve product quality. Examples of use cases include:
- Model by first intent
- In-silico design of drug and processes leading to reduced experimentation
- Hybrid modelling, rapidly correlate production parameters with quality attributes
- Zero touch regulatory filing
- Automated collection of data inputting directly into regulatory files
- Automated change control process
- Production control
- Automated continued process verification
- Process analytical technology for process control, maintain quality, and release product
- Prescriptive digital twin
- Paperless tech transfer
- Enterprise recipe management
- Single digital risk platform across enterprise
- Integrated project management system
- Automatic data transfer across enterprise
- Supply Chain
- Automated collection of regulatory, quality, pricing, and product info required for distribution
- End-to-end visibility
What Do Pharma Companies Need To Do To Enable The Change?
The jump toward data-led drug development will require significant changes in how pharma companies work compared with today’s processes. Deep-rooted ways of working and organizational structures and skills will need to change and evolve; furthermore, technology platforms across the value chain and throughout the technology stack will require significant integration so that data is accessible. These are the areas that pharma companies must change to achieve a sustainable data-driven organization.
- Platform – A significant challenge will be to implement an integrated platform. A common platform would connect all data across the disparate siloed systems and processes across the value chain, from R&D, CMC, and new product introduction (NPI) through to manufacturing. A common platform would also connect data from machine sensors, robots, and IoT devices throughout the technology stack, including the manufacturing execution systems, ERP, and quality management systems. The opportunity lies in the concept of a data fabric, by creating a logical and physical architecture.
- Data analytics organization and data analytics tools – The shift toward data-led design will include a change in skills across the organization. Pharma companies have already invested significantly and developed pockets of data analytics and data engineering capabilities, but this will need to scale further and become common practice across all the processes. Data management tools should harmonize, rationalize, and simplify how data is managed across the product life cycle, enabling experts to develop and scale out solutions and use cases quickly with standardized data pipelines that collect and transform data quickly.
- Processes and governance – Finally, the use of data will drive changes across business processes and governance. Processes that were once manual and reliant on paper will change. Data will automate processes and provide staff with the information they need in an instant and at the right detail, creating leaner processes that drive faster and more accurate decision-making that is less reliant on senior leadership to make all decisions.
Pharma companies will continue to use data to develop new products, but a more significant change is needed if new products will be designed and transferred across a more complex portfolio, seamlessly across the value chain. It will require a new way of thinking, working, and organizational structure to enable data to take the lead. But the potential value is significant both commercially for pharma companies and for mankind.
About The Authors:
George Marinos is a supply chain and operations expert at PA Consulting. He leads PA Consulting’s digital supply chain proposition and works with medtech companies to help them harness digital technologies and innovation to improve their supply chains and broader operations.
Mark Humphries is the chief scientific officer at PA Consulting. He has delivered some of PA Consulting’s most impactful scientific inventions – from new drug delivery devices, gene therapies, and point of care tests to biomaterials replacement for plastics, novel sensing and detection systems, and developing new manufacturing processes for advanced materials.