Preparing A Framework For Artificial Intelligence And Machine Learning Validation: A 3-Step Approach
By Peter Baker, Live Oak Quality Assurance, and Ulrich Köllish and Jennifer Roebber, GxP-CC GmbH
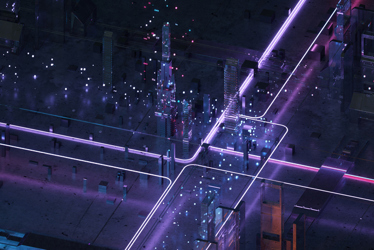
As the use of AI/ML in society has increased exponentially in the past few years, primarily because of increased availability and reduced costs of implementation, it is natural to expect its eventual and rather slow introduction into the GXP world. GXP users are generally slower to introduce new and disruptive technologies because of the heavily regulated nature of our business processes and low tolerance for risk/uncertainties. AI/ML has been used in drug discovery for some years and to some extent the GLP/GCP environments; however, it has not yet been adopted by the GMP commercial community.1
Recently, the FDA published a discussion paper on AI/ML in drug manufacturing1 and solicited feedback from industry. The industry’s comments2 show that an important factor preventing AI/ML implementation lies in a lack of regulatory guidance for the unique aspects of AI/ML not covered by existing computer system validation, such as, for example, minimizing bias in models, data protection, and quality for model training. Limited industry guidance, at least, has been proposed and outlined in the ISPE GAMP 5, second edition, published in July 2022.3
Earlier this year, FDA published two discussion papers1,4 in an attempt to gather industry’s views on additional use cases, implementation blockers, necessary data management activities for implementation, and other comments that may be useful as the agency develops more concrete guidance. Also, in 2021, the FDA's medical device division (CDRH) published AI/ML guidance,5 which we believe is much aligned with the existing pharmaceutical data integrity and risk management framework of subjectivity, formality, and decision-making. For example, the 10 guiding principles forming the Good Machine Learning Practice for Medical Device Development parallel the existing CDER guidance for three-stage process validation, published back in 2011.6 We expect that the guidance for pharmaceutical products will follow a similar path, with future guidelines echoing risk management within the existing cGMP framework.
In this article we discuss guidance already available that provides an excellent foundation for the eventual GMP AI/ML revolution, with the goal of preparing industry for future implementation while minimizing future growing pains. Waiting for further guidance to establish an AI/ML framework from the regulators to get started would represent a serious mistake, as the journey is a marathon rather than a sprint. As such, we propose taking action now via the following three steps:
Before we can begin to develop a pathway forward, we must remember the difference between qualification and validation. In the case of AI/ML, we would speak of qualification in the context of the model or platform itself, and validation as the use case in the context of the overall business process, including the process, the environment, and the operators.7 FDA, in its guide for data integrity, cites validation for intended use including “software, hardware, personnel and documentation.”8 Qualification provides the scientific evidence that the model functions appropriately within the Good Machine Learning Practice framework (yet to be defined by CDER, but likely following on the heels of the CDRH SaMD guidance9), while validation demonstrates that risks arising from the use of the model in a GXP environment are controlled according to its “intended performance.” Demonstrating either of these requirements during regulatory inspection is tricky for AI/ML and likely must rely heavily on risk management. The burden will be on the company to demonstrate how the use of the model within the validated process does not add unnecessary risk to patient safety (meaning better than the status quo). Considering this burden, a black-box defense will likely not be tolerated, as it does not mitigate the potential for risks such as biases in model performance.
Step 1: QRM And Intended Use
A solid quality risk management (QRM) framework within your network is essential, as the intended performance requirements of AI/ML will vary greatly depending on patient proximity, from upstream process control (generally lower risk) to downstream QA decision-making (higher risk). Experience using new tools, such as the informal/semi-formal and qualitative tools (e.g., data and process mapping) pushed in the new ICH Q9 revisions10 during routine legacy GMP operations, will pay dividends in the future, as it allows the SME to clearly explain the intended performance of the model during regulatory inspection, greatly reducing the potential for confusion and misunderstanding. In the same sense, quantitative and formal tools relying on multiplication of risk categorization impede critical thinking and will likely ultimately fail to produce the rationale necessary to fulfil the burden of intended use.
Step 2: Data Governance And Acknowledging Bias
In any industry adopting AI/ML applications, a solid data governance program is a prerequisite. No amount of good machine learning practices can address unknown biases in the data sets to be used for qualification and any future decision-making. Luckily, the road map to success for governance has been clearly outlined in the PIC/s guide for data management and integrity (design, operation, monitoring).11 Good data governance does not mean that bias is eliminated but rather acknowledged, reduced to an acceptable level (if necessary), and regularly monitored.
According to the State of MLOps Industry Report 2023, “over a quarter (27%) of ML practitioners surveyed believe that bias will never truly be removed from AI-enabled products.”12 So, it appears the goal is not to demonstrate freedom from bias (perfection) but rather to identify sources of bias and acknowledge limitations in the model output accordingly, which happens to be the same goal described under the PIC/s data governance guidelines.
In anticipation of AI/ML in future applications, we also recommend dedicating significant resources now to strengthen governance programs that go above and beyond minimum GMP requirements. Creating new roles within your organization such as a chief data officer to propose strategies for use of your data, assigning ownership and responsibility to data sets, and management’s promotion of an overall data culture will provide the governance foundation necessary to benefit from any future AI/ML use cases.
Step 3: Development Of Internal AI/ML Standards And Quality Oversight
In our recent experience, we have found that many companies are already well into qualification activities for AI/ML models, which is in-line with published use cases from natural language processing to QC data trending to digital twins.13,14 However, our conversations demonstrated that most sites are currently just beginning the process of establishing AI/ML frameworks within their quality system and developing as they go. By not preemptively establishing standards, these companies are making the future defense of the model for its intended use difficult as there is limited ability to recreate the qualification activities using scientific evidence (e.g., documentation). We foresee a difficult journey ahead if the quality unit is not involved from an early stage, implementing a standard set of expectations that must be followed.
We recommend implementing a good AI/ML standard prior to initiating development activities, so that any knowledge about the model gathered during early development can be referenced within the risk management program alongside some Quality oversight. As is well understood within the AI/ML community, one of the biggest risks is model bias, which comes in different forms and can arise at different points within the model development. We anticipate that the inability to produce documentation regarding the prevention of bias during model development will be a significant source of regulatory concern. As AI/ML is designed to drive future decisions regarding events that are likely to occur based on what the model “learned” from its training data, we have to demonstrate that the way the training data was collected and selected was unbiased – meaning free from influence that would cause the model to no longer meet fundamental scientific standards. An analogy can be drawn to human behavior: it is well understood that employee training in any particular activity is heavily influenced by any bias the trainer exhibits during the training activity, such as, for example, a dislike for a specific action outlined in the SOP. Demonstrating that the model is free of bias can be tricky and very high-risk – as evidenced by the many established case studies in society where bias in AI/ML has caused serious harm.15–17 On the bright side, once we develop QRM maturity, implement good risk-based governance, and roll out a standard framework with Quality oversight, AI/ML becomes feasible and, in our opinion, will greatly enhance the quality of medicines via multiple means yet to be discovered.
Conclusion
The potential applications of AI/ML in drug manufacturing are meaningful and will lead to reduced errors, recalls, and drug shortages. We see AI/ML as a needed tool to overcome current life-threatening situations and so much more than just a cool new technology. FDA’s Drug Shortage report from 2022 describes a dire situation with regard to access to essential medicines, with shortages increasing 30% as compared to 2021, with 62% of these shortages due to quality and manufacturing issues.18 Surely, new cGMP solutions are necessary and cannot wait – patients are waiting, literally. Our recent conversations with some in industry have been promising, and we are excited to see this transformation already underway. With a few minor additions to our existing quality system referencing existing regulatory guidance, we can be sure the future is bright.
References:
- Discussion Paper: Artificial Intelligence in Drug Manufacturing, Notice; Request for Information and Comments. Federal Register. Published March 1, 2023. Accessed July 10, 2023. https://www.federalregister.gov/documents/2023/03/01/2023-04206/discussion-paper-artificial-intelligence-in-drug-manufacturing-notice-request-for-information-and
- Regulations.gov. Accessed July 10, 2023. https://www.regulations.gov/document/FDA-2023-N-0743-0001/comment
- GAMP5-A Risk-Based Approach to Compliant GxP Computerized Systems, Second Edition.pdf.
- Using Artificial Intelligence and Machine Learning in the Development of Drug and Biological Products; Availability. Federal Register. Published May 11, 2023. Accessed July 10, 2023. https://www.federalregister.gov/documents/2023/05/11/2023-09985/using-artificial-intelligence-and-machine-learning-in-the-development-of-drug-and-biological
- Health C for D and R. Artificial Intelligence and Machine Learning in Software as a Medical Device. FDA. Published online September 27, 2022. Accessed July 10, 2023. https://www.fda.gov/medical-devices/software-medical-device-samd/artificial-intelligence-and-machine-learning-software-medical-device
- Guidance for Industry on Process Validation: General Principles and Practices; Availability. Federal Register. Published January 25, 2011. Accessed July 10, 2023. https://www.federalregister.gov/documents/2011/01/25/2011-1437/guidance-for-industry-on-process-validation-general-principles-and-practices-availability
- Pharmaceutical Inspection Convention Pharmaceutical Inspection Co-Operation Scheme. Good Practices for Computersed Systems in Regulated “GXP” Environments. Published online September 25, 2007. chrome-extension://efaidnbmnnnibpcajpcglclefindmkaj/https://picscheme.org/docview/3444
- Data Integrity and Compliance With Drug CGMP: Questions and Answers; Guidance for Industry; Availability. Federal Register. Published December 13, 2018. Accessed July 10, 2023. https://www.federalregister.gov/documents/2018/12/13/2018-26957/data-integrity-and-compliance-with-drug-cgmp-questions-and-answers-guidance-for-industry
- Software as a Medical Device: Clinical Evaluation; International Medical Device Regulators Forum; Guidance for Industry and Food and Drug Administration Staff; Availability. Federal Register. Published December 8, 2017. Accessed July 10, 2023. https://www.federalregister.gov/documents/2017/12/08/2017-26441/software-as-a-medical-device-clinical-evaluation-international-medical-device-regulators-forum
- EMA. ICH Q9 Quality risk management - Scientific guideline. European Medicines Agency. Published September 17, 2018. Accessed July 10, 2023. https://www.ema.europa.eu/en/ich-q9-quality-risk-management-scientific-guideline
- Pharmaceutical Inspection Convention Pharmaceutical Inspection Co-Operation Scheme. Good Practices for Data Management and Integrity in Regulated GMP/GDP Environments. Published online July 1, 2021. chrome-extension://efaidnbmnnnibpcajpcglclefindmkaj/https://picscheme.org/docview/4234
- iMerit. The 2023 State of MLOPS AI Goes Commercial. https://imerit.net/the-2023-state-of-mlops-report/
- Chapman J. Part 2: How Amgen Uses AI Tools To Improve Manufacturing Deviation Investigations. Redica Systems. Published September 18, 2019. Accessed July 10, 2023. https://redica.com/pharma-part-2-how-amgen-uses-ai-tools-to-improve-manufacturing-deviation-investigations/
- Paul D, Sanap G, Shenoy S, Kalyane D, Kalia K, Tekade RK. Artificial intelligence in drug discovery and development. Drug Discov Today. 2021;26(1):80-93. doi:10.1016/j.drudis.2020.10.010
- Obermeyer Z, Powers B, Vogeli C, Mullainathan S. Dissecting racial bias in an algorithm used to manage the health of populations. Science. 2019;366(6464):447-453. doi:10.1126/science.aax2342
- Klare BF, Burge MJ, Klontz JC, Vorder Bruegge RW, Jain AK. Face Recognition Performance: Role of Demographic Information. IEEE Transactions on Information Forensics and Security. 2012;7(6):1789-1801. doi:10.1109/TIFS.2012.2214212
- Caliskan A, Bryson JJ, Narayanan A. Semantics derived automatically from language corpora contain human-like biases. Science. 2017;356(6334):183-186. doi:10.1126/science.aal4230
- FDA. Drug Shortages CY 2022. chrome-extension://efaidnbmnnnibpcajpcglclefindmkaj/https://www.fda.gov/media/169302/download
About the Authors:
Peter E. Baker is president of Live Oak Quality Assurance LLC. He has been consulting nationally and internationally since 2019. Before consulting, Peter spent 11 years as a U.S. FDA drug investigator, with seven of those years in FDA’s overseas offices in India, China, and Chile. Peter was named FDA Investigator of the Year in 2013 for his work uncovering serious breaches in data integrity and has special interest in Big Data management and compliance with 21 CFR Part 11.10(a).
Ulrich Köllisch is an associate partner in GxP-CC, and his expertise lies in life sciences compliance and particular specialization in data integrity and audits. He has led data integrity initiatives and supported many organizations in the pharmaceutical and biotech sector executing data integrity campaigns. Ulrich has experience in consulting for audit preparation and conduction in the GMP and GCP areas, is co-heading a PDA special interest group, and is an active participant in other industry knowledge groups. Ulrich has a Ph.D. in physics in the area of medical imaging from the Technical University Munich.
Jennifer Roebber is a consultant at GXP-CC. She has nine years of research experience in diverse laboratory environments. She earned her Ph.D. from the University of Miami Miller School of Medicine. She worked as a visiting research associate at Cardiff University and later as a research scientist at the University Hospital of Wales studying sleep impairment among people with epilepsy.