Recent Explosion Of Start-Ups Underline Business Need For AI/ML In Drug Discovery, Development & Clinical Trials
By Adam Lohr, RSM US LLP
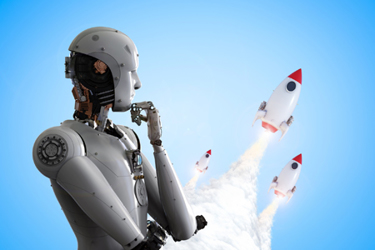
“Gradually, then suddenly” — this line from Ernest Hemingway’s The Sun Also Rises — embodies the impact that artificial intelligence (AI) and machine learning (ML) will have on our economy and the future of life sciences.
From doctor visits and fitness trackers to drug discovery and vaccine development, it is important to understand what AI and ML are, what they are not, and why companies may be leveraging these technologies in the future.
Adapting To A New Business Environment
Computational capabilities, ubiquitous data, and increasing competition for a highly skilled workforce are some of the practical forces behind the expansion of AI and ML in business applications. The reality is that businesses, including life sciences businesses, are becoming more digitized and are pursuing increasingly complex problems. AI and ML have the potential to enhance existing workforces and business processes and allow companies to do more with less in a globally competitive ecosystem.
The number of life sciences startups continues to increase, with an average of 1,000 companies receiving their first private equity or venture investment every year for the past seven years, according to RSM’s analysis of PitchBook data.
Source: PitchBook
While this influx of new companies and technologies is spurring innovation in the industry, these startups are increasingly in competition for talent and capital. Twenty years ago, first-time investments in life sciences companies represented 40% of the investments and 20% of the capital invested. In 2021, only 20% of the deals and 9% of private life sciences capital is flowing to first-time fundraisers. With that said, the entire investment pie continues to increase, and the last several years have seen record levels of private investment and initial public offering capital raised. This influx of well-financed companies has also led to a shortage of highly skilled workers and, according to labor data from the U.S. Bureau of Labor Statistics, hiring in life sciences is at record levels.
Based on analysis of companies that successfully launched their first commercial drug between January 2020 and June 2021, the average amount of capital raised between founding and Food and Drug Administration approval was $330 million. More remarkable is that six drugs received approval having raised $100 million or less.
Source: EvaluatePharma Ltd.
Noteworthy, too, an increasing number of newly approved drugs were the first commercial products for their sponsors. In 2020, 22% of new drugs were the sponsor’s first drug, and through June of 2021 that number has increased to 45%. Record levels of investment, new market entrants, and the rate of first-time launchers are all indications that life sciences companies are looking for new ways to achieve commercial success as efficiently as possible. This is where AI and ML begin to show their potential.
Working With The Machines
AI falls into the overarching field of computer science but encompasses a wide range of subsets — primarily deep learning and machine learning. In simple terms, artificial intelligence aims to create machines capable of thinking and acting like the human brain. Machine learning is the idea that given access to data sets and algorithms, these “machines” should be able to learn things about the data, like recognizing patterns and predicting outcomes. Going one step further, deep learning focuses on arranging algorithms in layers — known as “artificial neural networks” that are then able to learn and make decisions independently.
It is estimated that by 2025 our global datasphere will contain 175 zettabytes of information. It would take 1.8 billion years for the average computer to download that much data. With ML, we could begin to identify trends, patterns, and anomalies in a more efficient and global scale that we simply can’t do today. With these tools, collection and analysis of medical literature and patient data becomes faster and simpler and can be used to tailor drugs better suited to each individual patient.
Although a fairly new area with respect to the use of AI, the drug design and development process is ripe for the application of machine learning and deep learning techniques. With these techniques, researchers are able to run diagnostics on proteins, compounds, and experimental drugs and evaluate efficacy and potential problems before transitioning to clinical trials. Discovering potential defects and indications of efficacy at such an early stage can dramatically decrease development costs and timelines.
Fully utilizing the capabilities of AI is likely a decade or more off, but the computing and prediction capabilities of ML are more immediately realized. Because of the massive amounts of data collected in modern clinical trials, researchers are evaluating how ML can analyze historical data sets, patient data, and biobanks and apply that data in future clinical trials to reduce the rate of failure. Machine learning could be used to prevent the need for “rescue studies” and assist researchers in developing and designing studies that are more likely to be approved and less likely to fail. Although there is limited potential to solely use AI and ML to perform a given clinical trial, this method would allow researchers to get a rough test of how the drug might perform and to identify potential failures before the drug goes into trial.
A similar approach has been applied in the development of cancer treatment, making it easier to see what treatments might be most effective without actually engaging in trials. For several years, large-scale artificial intelligence projects have been used to suggest novel drug hypotheses for the treatment of cancers at a rate competitive with purely human research but at much greater speed. PubMed and similar systems work as repositories and search engines for medical publications and other data, boasting high throughput and precision in explaining and identifying cancer driving pathways. As explored by researchers at the University of Arizona and Oregon Health & Science University, these systems seek to show that “combining human-curated ‘big mechanisms’ with extracted ‘big data’ can lead to a causal, predictive understanding of cellular processes and unlock important downstream applications.”
Coronavirus: Catalyst For AI And ML In Drug Development
Artificial intelligence and the body of knowledge it encompasses have proven to be a powerful force in the global response to the novel coronavirus. The pandemic launched the life sciences industry to the forefront of scientific, social, and economic discussions with the world watching and waiting for a full-scale, effective, and immediate response. The understanding that a coronavirus vaccine would be our best bet to a timely return to "normalcy" intensified the pressure for an unprecedented acceleration of the SARS-CoV-2 vaccine development.
In a world where drug discovery and development are hindered by high failure rates, higher costs, and timelines that stretch years if not decades, technology proved to be a game changer. Within weeks of sequencing the virus’ genome, AI-powered technologies were being deployed to evaluate protein structures, sift through potential vaccine targets, and begin early-stage drug testing. In fact, before the genome sequencing had even been completed, researchers at the University of Basel in Switzerland had already begun using an AI-powered modeling tool to predict the structures of the proteins on the outer surface of the virus — those that are crucial to identify for vaccine design purposes.
Typically, drug discovery takes between three to five years, with researchers analyzing thousands of compounds as potential targets to test. However, in the case of COVID-19, the rapid sequencing of the genome, thanks in part to advancements in sequencing technologies, allowed scientists to begin understanding the virus’ structure within weeks. By the end of January 2020, the biotech firm Cyclica deployed a task force utilizing its AI-augmented drug discovery platform. Ligand Design used its deep learning tool, MatchMaker, to go through over 6,700 approved drugs and drug candidates with Phase 1 clinical trial data to identify possible drug repurposing opportunities.
Meanwhile, a team of computer scientists at the Stanford Institute for Human-centered Artificial Intelligence used machine learning in the form of linear-regression models together with neural-network algorithms to generate a list of targets on the coronavirus’ genome that were likely to provoke an immune response, signifying one of the first steps in vaccine development.
At BenevolentAI, their AI platform created a knowledge graph of potential drugs that could help block the viral infection process of COVID-19. Using these tools, they hypothesized that baricitinib, a drug used to treat rheumatoid arthritis, could reduce viral infectivity, replication, and the inflammatory response. By late November 2020, the FDA had approved emergency use of baricitinib for COVID-19 patients after the hypothesis was upheld in a randomized control trial.
Examples such as these underscore the power of technology, putting drug developers on an accelerated pace for vaccine development. Even after the initial COVID-19 vaccines were developed, the spread of variants pushed a team of researchers at the University of Southern California to develop an AI-powered model capable of constructing a multi-targeted vaccine for a new virus in less than a minute, with results validated in under an hour. This is a far cry from the traditional approach of discovery, development, and testing.
Addressing Challenges Throughout The Development Cycle
|
Discovery |
Development |
Clinical Trials (CTs) |
Challenges |
|
|
|
Why AI/ML fits |
|
|
|
State of adoption |
In practice |
Not yet in practice |
Limited deployment |
Intersection Of Public Health, Technology, And Regulatory Norms
While the technologies, platforms, and applications utilizing artificial intelligence have come a long way since the term was first coined in the mid-1950s, the political and regulatory framework surrounding the field is a bit more muddled. In recent years, there has been an exponential increase in the pursuit of rare diseases, which are a prime target for the use of AI powered drug discovery. For context, during the past eight years, the FDA has doubled its new drug approval rate for drugs combatting rare diseases, compared to the previous eight years. The FDA is also receiving and approving AI-based applications at record rates. In 2014, the FDA had approved only one AI application, which was for use in detection of atrial fibrillation; and by the end of 2019, more than 70 AI approvals had been granted. This indicates that there is both market demand and regulatory appetite for the advancement of AI and ML in medicine.
It should also be noted that the vast majority of drug developers and biopharma companies do not develop their own AI and ML technologies, and instead rely on third party platforms that are deployed in their operating environment. Whenever a third party or their platform are leveraged it is important to understand the risks, and with an AI-based solution, two of those primary risks are its need to access vast amounts of data and likely intellectual property, as well as the potential impact that such solutions can have on a company’s decision-making. While AI products are submitted to the FDA for approval, they are not reviewed in the same manner as new drugs, where trial data is shared publicly and reviewed among regulatory panels. Instead, the majority of AI products are submitted for approval via the 501(k) pathway, which only requires “substantial equivalence” be shown to approved tools, rather than concrete evidence of a tool improving care. Additionally, there are no regulations or guidelines around AI tool developers and how they need to document how their AI was developed or whether or not it was tested on an independent data set. Since the algorithms’ results are only as diverse and comprehensive as the data they are fed, it makes it difficult to determine whether or not unintended consequences, biases, or falsities may be present in the tools’ findings. STAT reported that of the 161 AI products approved by the FDA, only 73 studies disclosed the patient data used to evaluate their devices. Of those 73 that reported patient data, only 13 disclosed a gender breakdown of the patients studied, and only seven disclosed the racial identity of their study participants.
These points of course bring up ethical, societal, and privacy concerns. There is limited regulation not only in documenting how these AI algorithms are developed and tested but also in how the data gathered is in turn collected and distributed. One area that the Health Insurance Portability and Accountability Act (HIPPA) fails to regulate is the area of retail genetic testing offered by firms such as Ancestry and 23andMe. Companies operating within this space can store user data for up to 10 years and can legally sell the data to pharmaceutical and biotech firms. In 2017, 23andMe received regulatory approval to analyze customers’ genetic data, scanning for risk factors for certain diseases such as Parkinson’s and Alzheimer’s, without consent from those customers. Examples such as these underscore the inconsistent nature of regulations within this space. It is clear that the very basis that these technologies operate on— patient data — must be adequately collected, used, and protected to ensure comprehensive, unbiased, and accurate results.
Faster And Cheaper Drug Development, Personalized Medicine, Better Patient Outcomes
Ultimately, the cost and scarcity of skilled labor to conduct traditional drug development has found its counterpoint in the speed and capabilities of Big Data, modern processing power, and the emergence of AI and ML.
The relative cost effectiveness and impressive capabilities of AI and ML within life sciences and healthcare give basis for why more and more companies have adopted and developed AI platforms and tools. The technology’s ability to recognize patterns and detect changes across wide arrays of data, including analyzing a wide scope of patient data, make it a suitable and ideal investment.
The application of artificial intelligence and machine learning in the development and discovery of drugs, as well as in pharmaceutical production and clinical trials, has been shown to lead to improved outcomes and decreased expense on human capital. Deep learning can be particularly effective in select applications with respect to outcomes and data.
Moreover, the ability of AI and ML in predictive analysis, including predicting patient outcomes in clinical trials, is leading toward advancements in precision and personalized medicine. This technology has the potential to move us away from just treating the symptoms of a disease to identifying, targeting, and analyzing the underlying cause, providing better treatment plans, more successful patient outcomes, and a more complete approach to healthcare. Further, the potential of AI to reduce the high costs of drug discovery and development, and the opportunity it presents to more thoroughly identify and manage drug repurposing opportunities, are contributing factors to AI revolutionizing the life sciences industry and the nature of healthcare as we know it.
About The Author:
Adam Lohr is an audit partner and life sciences senior analyst at RSM US LLP. In addition to providing assurance services to his clients, he also sits on RSM’s national life sciences team and leads the San Diego office life sciences practice. His senior analyst responsibilities include advising the firm’s life sciences clients and client servers as they work to navigate the rapidly changing industry environment. Adam regularly writes, presents, and advises on capital markets, digital transformation, policy, and other issues transforming the life sciences. He focuses on high-growth companies that are globally active in the life sciences, technology, and consumer products industries. He specializes in providing financial audit services and helping clients respond to technical, regulatory, and economic changes that impact their business.