The 5 Basic Tenets Of Data Integrity — And How Failures Occur
By Joanna Gallant, owner/president, Joanna Gallant Training Associates, LLC
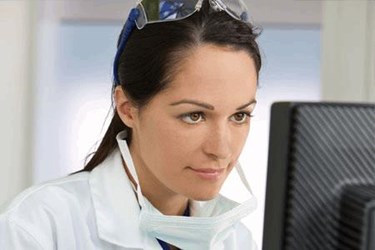
It amazes me that, with all the data integrity issues highlighted in regulatory agency inspections over the last several years, very few people I talk with today are familiar with the court case that set the legal precedent for data integrity standards. To me, this is one of the reasons why we see so many data integrity issues — those who forget the past are condemned to repeat it.
A Quick Summary Of U.S. vs. Barr Laboratories
In a nutshell, during the years leading up to the 1992 court case, FDA and Barr Laboratories disagreed on what constituted acceptable data handling practices under GMP. FDA felt that Barr Labs’ control practices — including release of product not meeting specifications, inadequate investigations of failed product, failure to control product manufacturing steps, and averaging of testing results — were not sufficient to ensure that products meeting its quality standards were distributed to the public. So on behalf of the FDA, the U.S. Attorney’s Office filed suit against Barr Laboratories.
The company’s argument, though, was that the practices FDA claimed it needed to follow were not specifically required by the GMPs. In response, Barr sued FDA for practicing “ad hoc” drug regulation.
The two cases were combined and heard by Judge Alfred Wolin, and it is Judge Wolin’s landmark decision that became the stake in the ground for current data integrity expectations in the GMP industries.
Much of the Barr Decision related to data management practices in quality control (QC) laboratories and became the basis of the FDA guidance Investigation of Out of Specification Results in Pharmaceutical Production (OOS guidance). But don’t be fooled into thinking the decision only applies to the QC laboratories, because when viewed from a higher level, the points drive controls for data generated across pharmaceutical companies.
What Are The Basic Principles Of Data Integrity And GMP Data Control?
While this article is not intended to be a complete summary of Judge Wolin’s decision and all that it discussed, let’s look at some of the data integrity principles the decision contained.
1. Processes work must work consistently.
First and foremost, we need to know that we are able to generate good data — which means our process must be able to reliably produce data we can trust. So, we must define and validate our processes and laboratory test methods to ensure reproducibility.
Then, once the process works, we need to maintain that process so it can continue to work as expected. Cleaning ensures nothing is added to the process, from residues or cross-contamination. Performing maintenance and calibrations ensures that equipment works correctly and is capable of continually producing good quality data.
And we must have data to support the cleaning process, the frequency of maintenance and calibration, and our claims that our process is reproducible. Without the data, we don’t have an argument that our processes are capable of functioning as expected.
2. We must always follow a written procedure.
In addition to making sure processes work consistently through validation, there are two other components to consistency: One is having the process documented in sufficient detail to allow for reproducibility, and the other is ensuring that documented process is followed every time. As simple as it may seem, we get in trouble here all the time.
In some cases, including those highlighted in 483 and warning letters, procedures do not include the level of detail that allows for consistent performance. Variability in performance equals variability in output and, subsequently, in results. As an example, consider this observation from a 483 issued in August 2013:
Written procedures … for cleaning manufacturing equipment do not always have descriptions in sufficient detail of methods, equipment and parameters (such as volume of water, time, pressure) used to ensure controlled, effective and consistent/reproducible cleaning results for a validated process. For example, the instructions read in part “Visually inspect the load for cleanliness at the completion of the cycle. If the equipment is not clean, then repeat the cleaning process.” Whereas in a validated cleaning process, delivering established and proven process parameters render equipment clean. But in this case, equipment follows the subjective decision making of the operator and is not controlled by the process itself. Another example is (), “Rinse all valve parts with water”. But no parameters such as volume of water, time, water pressure or equipment used are given.1
We tend to be an industry of scientists, and part of science is testing and tweaking, but we can’t do that in the QC laboratory and expect to generate data with integrity. Laboratory test methods must be validated and proven to be reproducible across analysts and instruments, specific enough to detect compounds and impurities in our products, and sensitive and accurate enough to identify and quantify these materials to the appropriate levels — which then also means that these methods can be sensitive to even minor variations in technique between analysts. Analyst-to-analyst variations are then compounded by on-the-fly changes, if made.
We need to impress upon people that our processes and methods must be in writing, they must be followed as written, and any errors or anomalies (including OOS, out of trend, or aberrant results) must be documented and assessed for impact. This encompasses not just our testing and production processes, but also our investigation process for those anomalies. Per the FDA’s OOS guidance, OOS investigations should occur using a predefined procedure — the procedure defines a standard process but still allows for the appropriate scientific experimentation as needed in the appropriate parts of the process to identify the cause of the anomaly.
3. All data must always be recorded as part of the official record.
And then, in accordance with written procedures, all data generated must be captured and documented appropriately — even data that is invalidated due to known errors. Without it, we lack a complete trail of our activities and decisions for each batch of product produced and tested.
Warning letter and 483 observations describe situations of test or trial injections being run outside the validated test method, along with examples of disposal or deletion of failing results, including through the use of systems in which the audit trails have been shut off. These situations are viewed as unacceptable because data is being generated unofficially, or official data is being discarded, versus being part of the story the testing is designed to tell.
This isn’t simply a QC laboratory problem, though. Similar situations can also occur in manufacturing, as well, where information isn’t documented, batch records don’t include thorough information, information is recorded on scrap paper, and more.
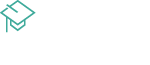
Joanna Gallant will host a live seminar on the proper handling of laboratory data/results — especially failing or out of specification (OOS) data:
Laboratory Data Integrity: Current Expectations for OOS Result Investigations
July 20, 2016 | 1:00-2:30PM EDT
4. Error cannot be assumed – it must be specified and supported with evidence.
The U.S. vs. Barr Labs case resulted in some specific definitions regarding “errors.” One definition says “laboratory error” occurs when an analyst makes a mistake in following the method/procedure, uses incorrect standards, or makes a miscalculation/mismeasurement. This definition can expand to include manufacturing operators or anyone else to whom similar situations would apply. Another definition of “non-process related errors” was put forth and includes those situations that occur when equipment malfunctions, humans err as indicated above, or other similar situations transpire in which something goes wrong due to an anomaly.
However, “process failures” are inherent issues in the process (like an incorrect mixing time or an incorrect weight documented in the procedure). These are not “errors” as in the previous sense, because the problem would have happened even if the operator followed the procedure and the equipment functions properly. They result from flaws in the process as it has been designed or documented.
Definitions aside, any error or failure situation must be investigated to identify the cause that triggered the issue. We can’t assume a mistake occurred simply because a person was involved; we must determine and provide evidence of that mistake, if it did in fact happen. We also can’t assume that a failure resulted from a mistake; it may indicate something went wrong in our process, and as a result, it can’t be discounted and must be investigated.
This is one reason why frequently citing human error as the root cause in investigations is a concern — it effectively says that a thorough investigation of the problem didn’t occur. (See the article Human Error Is The Leading Cause Of GMP Deviations – Or Is It? for a more thorough discussion of identifying root cause when issues appear to be human errors.)
5. Documentation describing each step of the process, and associated investigations, must be preserved.
At heart, data is evidence — proof that we executed procedures as required and, in doing so, produced good quality product. And we have to be able to trust the quality assessment our data provides, meaning there can be no holes in the story it tells. This includes appropriate justification for invalidating data, providing scientific rationale for decisions made and logical representation of results — even those that fail.
For example, we can’t change, batch to batch, how we report a set of results that includes a legitimate failure to show that the batch, on average, met quality standards. If any one result in the calculation is a failure, the calculation must reflect it and designate that the batch as a whole contains a failure. Something that may appear as an outlier may be a true failure. This is one reason why the MHRA’s OOS guidance does not allow for the use of outliers to disregard a valid data point. And when we retest, we must report all of the results generated in the process of testing/retesting, unless a legitimate error allows for a specific data set to be invalidated.
Then, we must tell a complete story of what was done, including all of the data generated, the decisions made and what they were based on, decisions made about the batch based on all of the available data, and then what was done to resolve any specific problems or issues that the situation identified. It all must be documented and retained, because without documentation, we can’t support the story.
What Are The Most Common Data Integrity Issues — And Where Can We Expect To Find Them?
So while the Barr Decision primarily spoke to controls around QC testing data, data integrity issues can occur anywhere in the data generation or control process (see Figure 1), anywhere in the company where data is generated — not just in the laboratories, even though the QC lab is where we hear about most issues being identified.
Figure 1: Data generation and control process
Common problems seen in each portion of the process that can contribute to data integrity issues include:
1. Personnel qualification:
- Not properly preparing personnel to generate or review data, including not requiring a competency demonstration prior to allowing independent task performance
- Personnel using improper techniques due to inadequate training, lack of oversight, or technique slippage
- Management focusing on throughput over accuracy/integrity of data
- Personnel engaging in active fraud/falsification activities
2. Task preparation and execution:
- Using unapproved suppliers (including CMOs/contract labs) or materials, which can introduce variation
- Accuracy/reliability issues with systems and equipment (including data acquisition and recording systems), such as failing to calibrate, validate, or provide appropriate access controls to the systems/equipment
- Not labeling materials, samples, or processing equipment appropriately, leading to errors and mix-ups
- Not providing enough detail in procedures to enable consistent performance, thorough data capture/collection, or appropriate verification/oversight
3. Data collection (including data capture, interpretation, and review steps):
- Inaccuracies between data capture systems and specification documents (i.e., programming/logic errors)
- Overburdened personnel not performing thorough reviews of data
- Changing approved records without reapproval/reverification to ascertain accuracy and process impact
- Mishandling, covering up, or failing to report deviations or OOS results
- Personnel documenting work that wasn’t completed
- Not following procedures, leading to inconsistency in data collection/interpretation
- Mislabeling or not labeling samples, materials, or processing equipment, introducing the possibility of data mix-ups
- Not completing or pre-completing documentation
4. Data maintenance and archiving:
- Access control problems, including sharing of passwords and providing unauthorized personnel access to edit/delete programming or data
- Failing to backup data or protect records from loss
- Altering, overwriting, or deleting failing data
- Hybrid systems lacking processes to manage/maintain both the paper and electronic components of the system
- Retention of raw data or of the complete data acquired or generated during a process
- Problems with issuance, use or control of batch records, worksheets, and notebooks
In Summary: No “Testing Into Compliance”!
While testing generates the checks of the organization’s process, the data about the process as a whole is the organization’s lifeblood. And just like blood, when it’s poisoned, the whole system it supports is also poisoned.
To avoid this, we need to ensure we educate people on the expectations for data integrity and ensure our processes have the appropriate controls in place to protect against data integrity issues throughout the company. We’re testing FOR compliance, not INTO compliance.
Reference:
- FDA Form 483 issued to OSO BioPharmaceuticals Manufacturing, August 2013 (The 483 can be purchased at http://fdazilla.com/store/form483/1643045-20130823.)