Why You Should Consider AI-Powered Digital Twins For Smart Manufacturing
By Karthik Raj Konnaiyan and Hemadri Doma, Tolmar Inc.
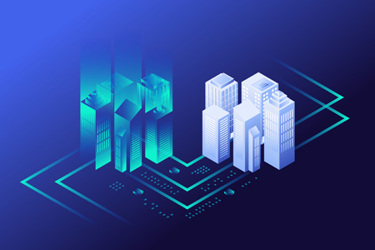
A digital twin is a digital representation of physical systems in the real world that is designed to simulate the components or the processes of those physical systems. Digital models of the systems are constructed based on data from multiple sources on the manufacturing floor. A digital twin model can react to the change in the input parameter and provide valuable information on the effect in the manufacturing process.1 The use of this data can range from optimizing the process parameters for better product quality to preventive maintenance of the physical components that are likely to break down. A digital twin can be used to run “what-if” conditions on the manufacturing floor without physically altering the process or the components of the systems. This makes the digital twin a powerful tool for smart manufacturing and a starting point to form the factory of the future. Figure 1 represents the concept of the digital twin in manufacturing.
Figure 1: Digital Twin in Manufacturing
How AI Can Enhance Digital Twin Benefits
Artificial Intelligence (AI) is revolutionizing industries such as manufacturing and healthcare by enhancing efficiency, automating complex tasks, uncovering hidden patterns in data, enabling real-time decision-making, and driving continuous innovation. In smart manufacturing, integrating AI with digital twin technology transforms production processes by enabling predictive analytics, real-time optimization, and autonomous decision-making.
The foundation of a digital twin lies in its ability to replicate a physical system using real-time data from IoT sensors, industrial control systems, and enterprise applications such as manufacturing execution systems (MES), enterprise resource planning (ERP), and laboratory information management systems (LIMS).2 AI plays a critical role in processing this vast amount of structured and unstructured data to identify inefficiencies, predict failures, and optimize system performance dynamically.
One of the key advantages of AI-powered digital twins is predictive maintenance. Machine learning models analyze sensor data, historical performance records, and operational conditions to predict equipment failures before they occur. This proactive approach minimizes unplanned downtime, reduces maintenance costs, and extends asset lifespan. AI-driven anomaly detection also helps identify deviations in production parameters, preventing defects and ensuring product quality in highly regulated industries such as drug manufacturing. Moreover, AI enhances process optimization by running real-time simulations to test different manufacturing scenarios under various conditions. Reinforcement learning algorithms can recommend optimal process adjustments to maximize yield, minimize energy consumption, and improve resource utilization. This capability is particularly valuable in industries that require stringent process controls, such as biopharmaceuticals, where variations in environmental conditions can impact product efficacy.
By leveraging AI-driven digital twins, manufacturers can transition from reactive to predictive and prescriptive operations, enabling smart factories with self-optimizing systems that improve efficiency, reduce waste, and accelerate innovation. This integration paves the way for a more agile, resilient, and sustainable manufacturing ecosystem aligned with the principles of Industry 4.0 and Pharma 4.0.
Figure 2: Digital Twin with AI Enhancements
In scenario planning and risk assessment, AI-based digital twins simulate manufacturing environments, supply chain disruptions, and clinical trial conditions to predict potential risks and recommend mitigation strategies. This allows pharmaceutical companies to optimize process parameters, ensure regulatory compliance, and proactively adjust logistics to prevent drug shortages. AI-based digital twins integrated with manufacturing execution systems (MES) can ensure precise formulation, adaptive dosing, and continuous quality monitoring that will guide from mass production to precision-based therapies.
In biopharmaceutical manufacturing, AI-driven digital twins enable use of tools like scGPT3 for real-time monitoring, predictive analytics, and optimization of bioprocesses by leveraging single-cell transcriptomics to enhance process understanding, detect anomalies, and improve yield and product quality.
By integrating AI with digital twin technology, the pharmaceutical industry can reduce drug development timelines, enhance treatment personalization, and improve manufacturing efficiency. This convergence of AI and digital twins is driving a shift toward data-driven, intelligent, and highly adaptive pharmaceutical and healthcare ecosystems.
Regulatory Considerations/Compliance
The integration of digital twins with AI in pharmaceutical manufacturing must align with Good Manufacturing Practices (GMP) and regulatory requirements based on the target market. Key regulations include FDA 21 CFR Part 11 (U.S.) for electronic records and signatures, EU GMP Annex 11 for computerized systems, and ICH Q7 for active pharmaceutical ingredients. Ensuring data integrity and security follows ALCOA+ principles, emphasizing accuracy, completeness, and traceability. AI and digital twin systems require validation under 21 CFR 820.70(i) and, where applicable, 21 CFR 211.68 for automatic, mechanical, and electronic equipment, or other types of equipment, including computers or related systems; and ISO 13485 for medical device quality management.
Best practices include rigorous model validation, real-time monitoring, continuous improvement based on periodic review, new data and feedback, robust data governance, and structured change management. Transparency in AI decision-making, ongoing improvements, and compliance with evolving regulations are critical. A risk-based approach, including failure mode and effects analysis (FMEA) and different risk assessment methodologies help mitigate potential failures. Ethical considerations, including bias prevention and data privacy, must be upheld by GDPR and other applicable laws. Proper training, documentation, audit trails, and collaboration with regulators ensure sustained compliance and operational excellence in AI-driven pharma manufacturing.
Future Outlook
The future of AI-powered digital twins in smart manufacturing will be driven by advanced AI agents, real-time monitoring, and next-generation virtual twin technology that enables autonomous, intelligent decision-making. AI agents will act as self-learning systems capable of analyzing vast datasets, predicting failures, and autonomously adjusting operations to optimize efficiency. Real-time monitoring and optimization will be enhanced with AI-driven predictive analytics, allowing manufacturers to detect deviations instantly and implement corrective measures without human intervention.
Figure 3: Future Outlook of Digital Twin in Smart Manufacturing
Virtual twin technology will create highly accurate, interactive 3D models of physical systems, enabling manufacturers to simulate different production scenarios and test optimizations before implementing them in real-world environments.4 The emergence of quantum digital twins, powered by quantum computing, will take simulations to unprecedented levels by processing complex multi-variable optimizations at speeds unattainable by classical computing, revolutionizing materials science, process design, and supply chain logistics.5 These advancements will pave the way for lights-out manufacturing, where factories operate with minimal to no human intervention, relying entirely on AI-driven automation, robotics, and smart decision-making systems to enhance productivity, reduce waste, and achieve near-zero downtime. As AI and digital twin technologies continue to evolve, they will drive the next wave of hyper-efficient, self-optimizing, and fully autonomous smart factories, marking a paradigm shift in global manufacturing.
References
- R. Argolini, F. Bonalumi, J. Deichmann, S. Pellegrinelli, 31 July 2023. [Online]. Available: https://www.mckinsey.com/industries/industrials-and-electronics/our-insights/digital-twins-the-key-to-smart-product-development.
- Kampa, "Modeling and Simulation of a Digital Twin of a Production System for Industry 4.0 with Work-in-Process Synchronization," Applied Sciences, 2023.
- H. Cui, C. Wang, H. Maan, K. Pang, F. Luo, N. Duan & B. Wang, "scGPT: toward building a foundation model for single-cell multi-omics using generative AI," Nature Methods, p. 1470–1480, 2024.
- C. Zhuang, J. Liu & H. Xiong, "Digital twin-based smart production management and control framework for the complex product assembly shop-floor," The International Journal of Advanced Manufacturing Technology , p. 1149–1163, 2018.
- D. O'Shea, 1 Aug 2022. [Online]. Available: https://www.insidequantumtechnology.com/news-archive/bosch-taps-multiverse-to-research-quantum-impact-on-digital-twins-2/.
About The Authors:
Karthik Raj Konnaiyan is the senior laboratory automation engineer at Tolmar Inc. He has expertise in digital health technologies, digital transformation in the pharmaceutical industry, pharma 4.0, and software as a medical device (SaMD). With a strong background in AI-driven process optimization, lab automation, and enterprise system integration, he has led transformative initiatives in pharmaceutical manufacturing and the medical device industry. He is focusing on advancing AI-based continued process verification (CPV), regulatory-compliant software modernization, and seamless data integration across laboratory and enterprise systems.
Hemadri Doma is a seasoned life sciences professional with more than nine years of expertise in the pharmaceutical and medical device industry. He is a subject matter expert in computer systems validation (CSV), computer software assurance (CSA), data integrity, equipment validation, process automation, artificial intelligence, pattern recognition techniques, and facilities validation. He has served in roles spanning engineering, facilities, information technology (IT), QC laboratory systems, process automation, validation, and quality processes. Doma currently holds the position of QA computer system validation engineer III at Tolmar Inc.